- Why ScienceLogic
- Main Menu
- Why ScienceLogic
Why ScienceLogic
See why our AI Platform fuels innovation for top-tier organizations.
- Why ScienceLogic
- Customer Enablement
- Trust Center
- Technology Partners
- Pricing
- Contact Us
- Product ToursSee ScienceLogic in actionTake a Tour
Experience the platform and use cases first-hand.
- Platform
- Main Menu
- Platform
Platform
Simplified. Modular-based. Efficient. AI-Enabled.
- Platform Modules
- Core Technologies
- Platform Overview
- Virtual ExperienceSkylar AI RoadmapRegister Today
Learn about our game-changing AI innovations! Join this virtual experience with our CEO, Dave Link and our Chief Product Officer, Mike Nappi.
November 26
- Solutions
- Main Menu
- Solutions
Solutions
From automating workflows to reducing MTTR, there's a solution for your use case.
- By Industry
- By Use Case
- By Initiative
- Explore All Solutions
- Survey ResultsThe Future of AI in IT OperationsGet the Results
What’s holding organizations back from implementing automation and AI in their IT operations?
- Learn
- Main Menu
- Learn
Learn
Catalyze and automate essential operations throughout the organization with these insights.
- Blog
- Community
- Resources
- Events
- Podcasts
- Platform Tours
- Customer Success Stories
- Training & Certification
- Explore All Resources
- 157% Return on InvestmentForrester TEI ReportRead the Report
Forrester examined four enterprises running large, complex IT estates to see the results of an investment in ScienceLogic’s SL1 AIOps platform.
- Company
- Main Menu
- Company
Company
We’re on a mission to make your IT team’s lives easier and your customers happier.
- About Us
- Careers
- Newsroom
- Leadership
- Contact Us
- Virtual Event2024 Innovators Awards SpotlightRegister Now
Save your seat for our upcoming PowerHour session on November 20th.
News Roundup, Feb 17, What’s Happening in AIOps, Observability, and ITOps
On this day in 1936, the world's first superhero, The Phantom, made his debut in comics. The Phantom’s creator, Lee Falk, got the inspiration for this purple crime fighter from historical and mythological legends like Zorro, Tarzan, King Arthur, and Robin Hood. Save the day for your organization by incorporating the latest trends in AIOps, observability, and ITOps into your company.
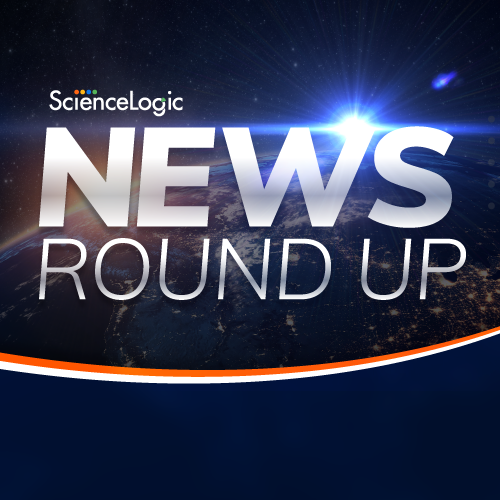
1.) Learn more about what observability is and isn’t from this article in The New Stack.
The way we design, build, and deliver software in the enterprise radically shifted in the move to microservices, containers and the cloud — what’s coined as “cloud native.” Another Digital Enterprise Journal survey reported that 58% of organizations lost visibility on the path from pull request to production after modernization projects.
To be precise, observability is a system trait, a descriptor, an adjective, not a telemetry checklist, and the boundaries between the data types are fuzzier than you may realize. Observability is how well someone can understand their system by using signals generated by instrumentation. Observability is not monitoring, and engineers are feeling the pain of status quo implementations. More than the telemetry you emit, and even the tooling you use, it is about including and empowering the human operator in the loop and a holistic telemetry strategy that can combat the high cost of telemetry at scale.
2.) Find out why observability is the perfect match for DataOps in this blog by InfoWorld.
Data management and DataOps teams spend significant effort building and supporting data lakes and data warehouses. Ideally, they are fed by real-time data streams, data integration platforms, or API integrations, but many organizations still have data processing scripts and manual workflows that should be on the data debt list. Unfortunately, the robustness of the data pipelines is sometimes an afterthought, and DataOps teams are often reactive in addressing source, pipeline, and quality issues in their data integrations.
Today, there are far more robust tools than Unix commands to implement observability into data pipelines. One aspect of DataOps observability is operations: reliability and on-time delivery from source to data management platform to consumption. A second concern is data quality. So DataOps and thus data observability must have capabilities that appeal to coders who consume APIs and develop robust, real-time data pipelines. But non-coders also need data quality and troubleshooting tools to work with their data prep and visualization efforts.
We’ve come a long way from using Unix commands to parse log files for data integration issues. Today’s data observability tools are a lot more sophisticated but providing the business with reliable data pipelines and high-quality data processing remains a challenge for many organizations. Accept the challenge and partner with business leaders on an agile and incremental implementation because data visualizations and ML models built on untrustworthy data can lead to erroneous and potentially harmful decisions.
3.) Forbes explains how large language models (LLMs) like ChatGPT are accelerating AIOps.
AIOps is an exciting area where artificial intelligence is leveraged to automate infrastructure operations and DevOps. It reduces the number of incidents through proactive monitoring and remediation. Public cloud providers and large-scale data center operators are already implementing AIOps to reduce their cost of operations.
One of the classic use cases of AIOps is the proactive scaling of elastic infrastructure. Instead of constantly monitoring the CPU or RAM utilization to trigger an auto-scale event, a deep learning model gets trained on a dataset representing the timeline, the inbound traffic, and the number of compute instances serving the application. The model then predicts the optimal capacity. The shift from reactive to proactive scaling has saved thousands of dollars for retail companies with consumer-facing websites.
The power of AIOps lies in its ability to automate the functions typically performed by DevOps engineers and Site Reliability Engineers (SRE). It will significantly improve the CI/CD pipelines implemented for software deployment by intelligently monitoring the mission-critical workloads running in staging and production environments. Large Language Models (LLMs) such as GPT-3 from OpenAI will revolutionize software development, deployment, and observability, which is crucial for maintaining the uptime of workloads.
While GPT-3-based models such as Codex, GitHub Copilot and chatGPT assist developers and operators, the same GPT-3 model can come to the rescue of the SREs. An LLM model trained on logs emitted by popular open-source software can analyze and find anomalies that may lead to potential downtime. Combined with the observability stack, these models automate most of the actions a typical SRE performs. Observability companies like ScienceLogic have integrated machine learning into their stack. The promise of this integration is to bring self-healing of applications with minimal administrative intervention. Large Language Models and proven time-series analysis are set to redefine the functions of DevOps and SRE, playing a significant role in ensuring that the software running in the cloud and modern infrastructure is always available.
4.) Find out why cloud is of utmost importance for financial services undergoing digital transformation in this blog by MIT Technology Review.
For many organizations, digital transformation has meant shifting to cloud-based architectures, tools, and processes. Working in the cloud and building cloud-native applications means quicker release updates, rapid scalability due to distributed compute power, optimized cost structures, and access to specialized tools that simplify tasks like testing, monitoring, and security.
Alongside simplification, digital transformation is also driven by a need to provide better products for customers, while still addressing security vulnerabilities and regulatory requirements. Digital transformation does not necessarily mean older systems will be replaced; instead, many are well-suited to be adapted, and freed from their dependence on mainframes. Public cloud and cloud-native technology are increasingly used to renovate these systems, helping organizations eliminate technical debt, quicken development cycles, and modernize technology stacks.
The modern technology stacks of financial services companies must be highly secure. One way to demonstrate data security and compliance is with infrastructure as code (IaC), which provisions and manages infrastructure through code instead of through physical hardware configuration or with configuration tools. As organizations migrate to the cloud and adopt modern technologies—such as serverless cloud, containers, and Kubernetes—infrastructure must be monitored and secured at an increasingly granular level. IaC can provide the tools to accomplish this.
Want to learn more about AIOps? Read this eBook>