- Platform
- Main Menu
- Platform
- Platform Overview
- SL1
- Skylar AI
- Skylar Advisor
- Skylar Analytics
- Skylar Automated RCA
- Restorepoint
- Hybrid Cloud Monitoring
- Multi-Cloud Monitoring
- Network Monitoring
- Integrations
- Trust Center
- Technology Partners
- Why ScienceLogic
- Compare
- Virtual KeynoteWatch the Video
Introducing Skylar AI: the suite of advanced AI capabilities that will redefine productivity, creativity, and decision-making across industries.
- Solutions
- Main Menu
- Solutions
- By Industry
- Solutions
- By Industry
- Enterprise IT Solutions
- Global System Integrators
- Service Providers
- Government & Public Sector
- Financial Services
- Channel Partners
- Learn More
We saw a better than 80% reduction in incident-related noise.
Download the Forrester Total Economic Impact™ which examined four enterprises with large, complex IT estates to measure the value and return on investment of ScienceLogic's AIOps Solution.
- By Solution
- Solutions
- By Solution
- AIOps Digital Transformation
- Business Service Management
- Tool Consolidation & Modernization
- IT Workflow Automation
- IT Infrastructure Monitoring
- Network Management
- Network Compliance
- Learn More
We saw a better than 80% reduction in incident-related noise.
Download the Forrester Total Economic Impact™ which examined four enterprises with large, complex IT estates to measure the value and return on investment of ScienceLogic's AIOps Solution.
- By Use Case
- Solutions
- By Use Case
- Accelerate Incident Response with Automated ITSM Workflows
- Automated Troubleshooting & Remediation
- Eliminate Visibility Gaps with Hybrid Cloud Monitoring
- Automate PCI DSS Compliance Checks for Network Devices
- Learn More
We saw a better than 80% reduction in incident-related noise.
Download the Forrester Total Economic Impact™ which examined four enterprises with large, complex IT estates to measure the value and return on investment of ScienceLogic's AIOps Solution.
- Customers
- Resources
- About
Data Lake vs Data Warehouse: The Future & Past of IT Operations
What are the differences between a data warehouse and a data lake? Are they the same? How are they different? Do I need one? Do I already have one?
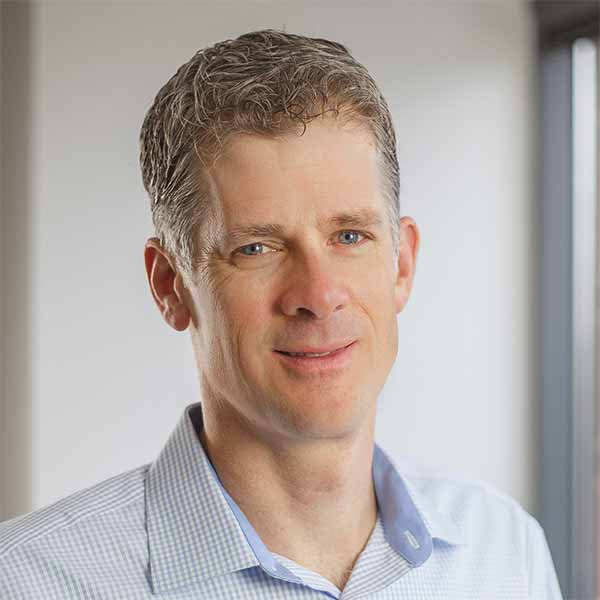
It’s not uncommon for kindred terminology to be used in high technology in data science, which can make things confusing when new terms are coined or become relevant to an organization. So, I wanted to address a few questions related to data lake versus data warehouse to help clear things up.
Data Lake vs Data Warehouse
Data warehouses and data lakes are both widely used for storing data, but they are not interchangeable terms when it comes to cloud data. A data warehouse is not a data lake. Nor is a data lake a new sort of data warehouse. When it comes to comparing a data lake vs data warehouse, it’s important to keep in mind that data warehouses are the product of a different time in IT. Although still in use today, data warehouses do not align with the complex needs of operating today’s modern enterprises in data management.
A data warehouse is a big repository for specific types of structured and filtered data—collected over time and for a specific purpose. Its function is typically more about archiving and historical analysis, and less about operational resiliency. Data warehouses are often siloed, and the data collected and stored in them is used for a specific purpose where the value of that data increases over time to enrich the organization’s understanding of that certain purpose. Data warehouses are useful for spotting long-term trends and developing business intelligence where there is no sense of urgency behind the decisions being made through the insights gained from their analysis in data management.
While data warehousing collects and stores specific types of structured and unstructured data for non-urgent analysis, data lakes are designed to collect, normalize, analyze, and use all the raw data generated by an organization’s IT enterprise, including structured, unstructured, and topological data. In IT, this includes data federated from all of an enterprise’s different infrastructure sources, like Google, Microsoft, and Amazon, as well as events, faults, logs, configurations, and performance.
While the raw data is useful in data science, what’s more valuable is a clean, normalized data lake wherein the raw data is organized in such a way that users can use the data for further queries or analysis. Normalization is the process by which data in the data lake is de-duplicated, formatted consistently, grouped logically, and stored in a more organized structure. Once normalized, the data can be visualized and analyzed. Without normalizations, a company can collect all the data it wants, but most of it will simply go unused, taking up space not benefitting the organization in any meaningful way.
The ScienceLogic Data Lake enables behavioral correlation of events and anomalies within a service context to determine the health, availability, and risk of business services. That gives real-time insights into the state of the infrastructure and applications that support the business. Those insights can then be used to determine the root cause of issues and automate IT processes and workflows in real time to resolve those issues. A clean data lake is also the foundation of achieving AIOps.
Data Lakes and the Three Vs
Artificial intelligence (AI) and machine learning (ML) are fundamental to AIOps and to the operation of data lakes—setting them apart from data warehouses. AI and ML are the keys to achieving the machine speed and accuracy needed to function effectively and efficiently in a modern IT environment. Data warehouses are big, slow siloes, whereas data lakes are an evolved concept for breaking down siloes and dealing with the “Three Vs” of big data: volume, variety, and velocity.
Accurate, consistent data is trusted data. Done right, a data lake provides the enterprise with a single source of trusted, dynamic data for managing all IT components and reducing the complexity of a “software-defined x” environment. Because the ephemeral systems and services today’s enterprises rely on produce a large volume of data, they need to be connected to a repository that can collect, process, and reason over that data as fast as it is needed. Then, those machine learning-powered insights are used to inform the decisions being made by the AIOps platform monitoring the flow of big data.
Large, complex IT environments include thousands of different data sources and data types, both structured and unstructured, and these must be aggregated into a single, consistent format that is used for IT infrastructure, application, and service-centric monitoring and management. A data lake offers real-time functionality to deal with the speed of change required to manage those complex IT environments. The process of collection, analysis, and use is a five-step, continuous cycle of collect, prepare, learn, analyze, and act. When a new service comes online, that data is immediately ingested into the data lake, normalized, and its relationships with devices and services both upstream and downstream are analyzed and put into effect for operations management.
Data Lakes and Customer Needs
That real-time collection, analysis, and use of all of an enterprise’s operational data is what sets a data lake apart from a data warehouse. A data lake is architected to support AIOps by solving for the complexity and variability of a multi-domain environment. This gives the enterprise nearly unlimited choice in building the kind of IT infrastructure it needs to operate at its most effective level. It does this by achieving comprehensive visibility across all of its IT estates, including on-premise legacy systems, virtual systems, and multi-vendor hybrid cloud environments.
After understanding the key differences between a data lake versus data warehouse, the question becomes, “how do I get one?” It’s the old build or buy debate, but where you may want to build and maintain your own data warehouse, a data lake is probably best left to buy as a part of your investment in AIOps. There are some things to keep in mind when making that decision, however, as there are costs associated with building a data lake.
An effective data lake provides analysis across the entire IT environment and that takes a combination of scalable storage and analytics, which needs to be engineered to be efficient or else it will be costly for the organization. It’s also important to prioritize speed over reliability, and while that seems counterintuitive, it is really more of a recognition that today’s enterprises are built with a great deal of resiliency, and so it is more important that your data lake be able to operate at a speed commensurate with the needs of your IT operation and the constant cycle of adds, moves, and changes.
Finally, you need to be able to get data from every configuration item (CI) in your enterprise from your data lake and into a configuration management database (CMDB). That means creating integrations for every technology that is part of your infrastructure. This is where the scales tips in favor of buy vs. build. Today’s customers don’t want to invest in new burdens for their IT operations teams. They don’t want to worry about the differences between a data lake and a data warehouse; they want to focus on running the business and forging partnerships that can manage the technical complexities for them and deliver a better user experience.
The ScienceLogic SL1 Data Lake
That’s where ScienceLogic comes in. Our AIOps platform, SL1, comes with its own data lake that is engineered with thousands of pre-built data integrations, as well as hundreds of additional pre-built, customizable PowerPacks to support integrations for the latest technologies as well as custom-built integrations specific to certain industries and scenarios.
If you’ve been wondering about the differences between data lake versus data warehouse, I hope this has cleared things up for you. And if you’re looking to transform your organization with an AIOps strategy, take a look at ScienceLogic’s SL1 platform and reach out with any other questions you might have.
Ready to learn more about AIOps and the SL1 Data Lake? Read this eBook»