- Why ScienceLogic
- Main Menu
- Why ScienceLogic
Why ScienceLogic
See why our AI Platform fuels innovation for top-tier organizations.
- Why ScienceLogic
- Customer Enablement
- Trust Center
- Technology Partners
- Pricing
- Contact Us
- Product ToursSee ScienceLogic in actionTake a Tour
Experience the platform and use cases first-hand.
- Platform
- Main Menu
- Platform
Platform
Simplified. Modular-based. Efficient. AI-Enabled.
- Platform Modules
- Core Technologies
- Platform Overview
- DemoExperience the Power of ScienceLogicRegister Now
See how today’s technology leaders are reducing costs, streamlining IT operations, and improving the customer experience.
- Solutions
- Main Menu
- Solutions
Solutions
From automating workflows to reducing MTTR, there's a solution for your use case.
- By Industry
- By Use Case
- By Initiative
- Explore All Solutions
- Survey ResultsThe Future of AI in IT OperationsGet the Results
What’s holding organizations back from implementing automation and AI in their IT operations?
- Learn
- Main Menu
- Learn
Learn
Catalyze and automate essential operations throughout the organization with these insights.
- Blog
- Community
- Resources
- Events
- Podcasts
- Platform Tours
- Customer Success Stories
- Training & Certification
- Explore All Resources
- WebinarThe Future of ITSM is AutonomousSign Up
Join our live webinar to hear how ScienceLogic and ServiceNow power intelligent service operations.
May 22, 2025
- Company
- Main Menu
- Company
Company
We’re on a mission to make your IT team’s lives easier and your customers happier.
- About Us
- Careers
- Newsroom
- Leadership
- Contact Us
- CongratulationsInnovators AwardsView the Winners
See how our winners have demonstrated exceptional creativity and set new standards in leveraging the ScienceLogic AI Platform to solve complex IT Ops challenges.
The Difference Between AI and Generative AI, and How ScienceLogic Uses Both
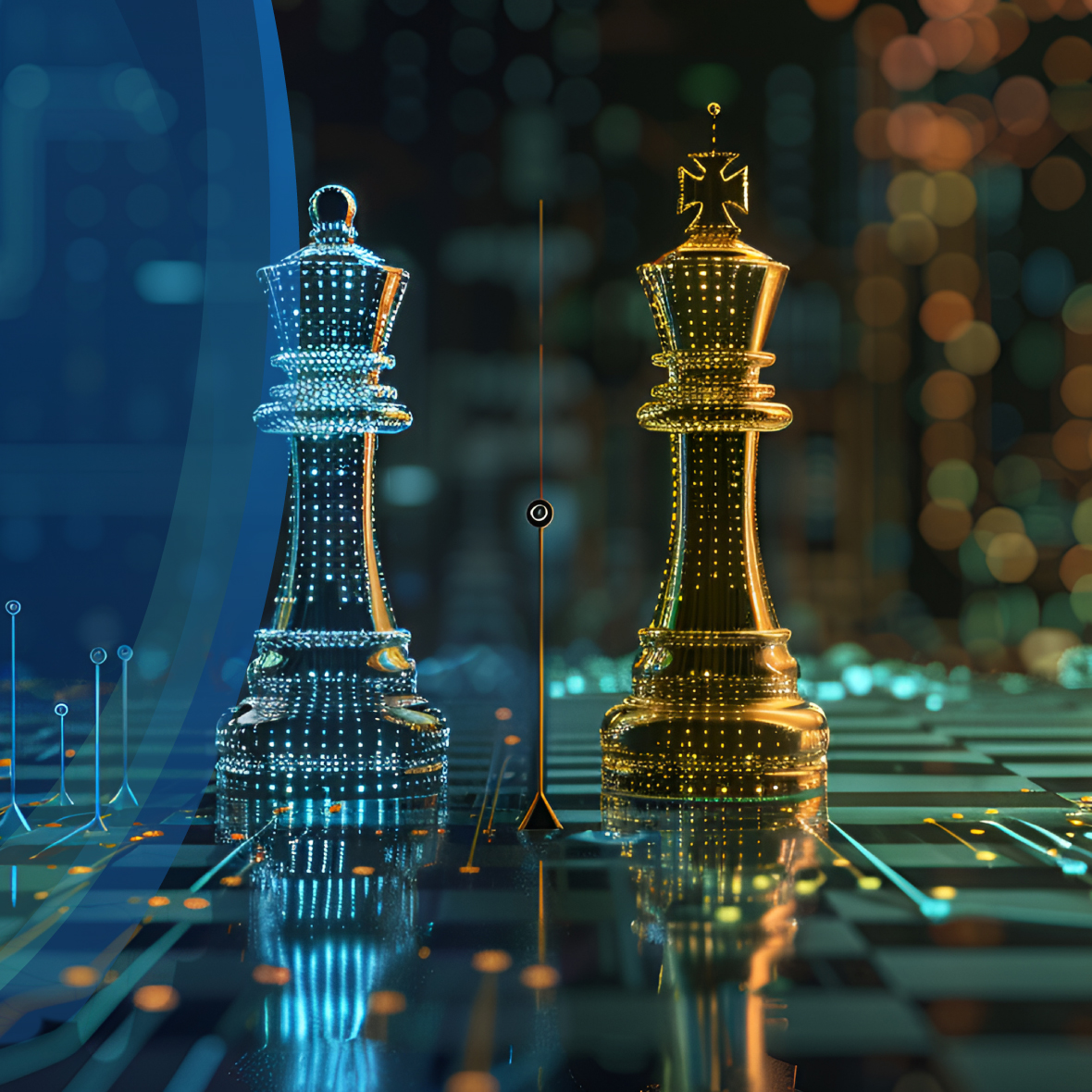
Artificial intelligence (AI) and generative AI – they may sound similar, but how do they differ? Although these terms are often used interchangeably, generative AI is actually a subtype of AI.
At ScienceLogic, we use many different types of AI, from machine learning to generative AI, to analyze IT environments, provide insights to help IT pros take action, automate and streamline workflows, and drive innovation.
There are many key differences between AI and generative AI, and how we use them to help organizations navigate the complexities of modern IT with confidence and deliver excellence at every turn of their digital journey.
Understanding Artificial Intelligence
AI has been studied and researched for decades. Gartner defines AI as applying “…advanced analysis and logic-based techniques, including machine learning (ML), to interpret events, support and automate decisions, and take actions.”
ML – a subset of AI – is an analytical discipline in which statistical models and algorithms are used to identify patterns and extract knowledge from data, much like a human would. However, ML can process data at a speed and volume far surpassing human capability. The insights ML derives are used to solve complex problems, make predictions, and power AI applications.
The better the quality of the data, the more effectively ML models learn, resulting in better outcomes.
Deep learning is a variant of ML. Modeled on the way the human brain works, deep learning uses multiple artificial neural networks to solve more complex problems. It is used in many ML and AI use cases, such as chatbots, autonomous vehicles, face recognition systems, and more.
Understanding Generative AI
Generative AI is a subfield of AI that focuses on creating content by learning patterns and relationships from large datasets. This type of AI can then create net new content that mimics the characteristics of the training data.
The most common example of generative AI is ChatGPT, where content (text, images, speech, video, etc.) is created in response to natural language requests or prompts from humans. Other use cases include AI assistants and copilots.
Gartner predicts that generative AI will “…become a general-purpose technology with an impact similar to that of the steam engine, electricity, and the internet.” We couldn’t agree more!
How ScienceLogic Uses AI and Generative AI
Since its inception, ScienceLogic’s vision has been to free up enterprise IT resources and optimize digital experiences – and AI and generative AI play a crucial role in this.
With the emergence of generative AI and LLMs, we’ve built on this foundation reimagining what IT can be with Skylar AI.
Skylar is a revolutionary suite of AI capabilities – including Skylar Automated RCA, Skylar Analytics, and Skylar Advisor – that empower IT teams to manage, anticipate, and prevent potential disruptions, ensuring continuous and efficient service delivery through curated and guided AI-first user experiences.
The Skylar AI suite includes powerful AI capabilities that help customers unify infrastructure monitoring, improve service visibility, accelerate time to resolution, automate ITSM and IT workflows, and focus on customer experience.
Here are some ways that ScienceLogic uses AI and GenAI in its Skylar AI suite:
- Identify root causes: Thanks to advances in generative AI and LLMs, we’ve improved our root cause analysis capabilities. Skylar Automated RCA uses unsupervised AI and ML (no manual training required) to quickly and automatically diagnose problems by analyzing logs in real-time. And because anything could break at any time, Skylar Automated RCA catches new problems, including unknown unknowns, before they cause incidents.
- Stay ahead of issues with predictive alerts: Current IT infrastructure monitoring systems rely on manual analysis to correlate service anomalies with underlying issues, requiring IT teams to spend time and resources troubleshooting. Instead, Skylar Analytics combines AI/ML analytics with deep data exploration and visualization to proactively uncover known and unknown issues before they impact service.
- Automate troubleshooting: Skylar AI automates complex troubleshooting tasks, saving hours (sometimes days) of manual effort and freeing up human experts to spend more time on innovation.
- Proactive, automated guidance to optimize IT operations: Skylar Advisor will go beyond today’s AI assistants. Using AI to analyze operational data, Skylar Advisor will provide tailored guidance and actionable, plain-language recommendations that help proactively avoid issues, optimize IT operations, and drive innovation without constant human expertise. The results will be guidance and suggestions in plain English that enable lower-level engineers to resolve issues as level 3 engineers focus on innovation.
How ScienceLogic Uses AI and Generative AI in the Journey to Autonomic IT
At ScienceLogic, we envision a world where AI becomes an indispensable partner in strategic planning, risk assessment, and opportunity identification, ultimately powering the future of autonomic systems with AI-powered innovation.
It’s a bold reimagining of IT that redefines IT monitoring and management and empowers organizations to journey to Autonomic IT.
As organizations progress on that journey, the focus shifts from traditional AI and the insights it provides to leveraging generative AI for innovation and efficiency.
But to recap our earlier definition of AI: AI outputs and AI-driven automation are only as good as the underlying data. The IT environment must be reconfigured to prioritize data quality and integrity so it can be synthesized from across IT operations and leveraged for machine-driven analysis, remediation, recommendations, and self-healing, self-optimizing IT ecosystems.
None of these capabilities would be possible without the global observability and quality data supported by the ScienceLogic AI platform.
Learn more about the Autonomic IT journey or contact us to determine your Autonomic IT maturity.
Accelerate Your Journey to Autonomic IT
Can your IT environment deliver the agility required to adapt to these demands – without increasing operational complexity or downtime? With Autonomic IT, it can.