- Why ScienceLogic
- Main Menu
- Why ScienceLogic
Why ScienceLogic
See why our AI Platform fuels innovation for top-tier organizations.
- Why ScienceLogic
- Customer Enablement
- Trust Center
- Technology Partners
- Pricing
- Contact Us
- Product ToursSee ScienceLogic in actionTake a Tour
Experience the platform and use cases first-hand.
- Platform
- Main Menu
- Platform
Platform
Simplified. Modular-based. Efficient. AI-Enabled.
- Platform Modules
- Core Technologies
- Platform Overview
- Virtual ExperienceSkylar AI RoadmapRegister Today
Learn about our game-changing AI innovations! Join this virtual experience with our CEO, Dave Link and our Chief Product Officer, Mike Nappi.
November 26
- Solutions
- Main Menu
- Solutions
Solutions
From automating workflows to reducing MTTR, there's a solution for your use case.
- By Industry
- By Use Case
- By Initiative
- Explore All Solutions
- Survey ResultsThe Future of AI in IT OperationsGet the Results
What’s holding organizations back from implementing automation and AI in their IT operations?
- Learn
- Main Menu
- Learn
Learn
Catalyze and automate essential operations throughout the organization with these insights.
- Blog
- Community
- Resources
- Events
- Podcasts
- Platform Tours
- Customer Success Stories
- Training & Certification
- Explore All Resources
- 157% Return on InvestmentForrester TEI ReportRead the Report
Forrester examined four enterprises running large, complex IT estates to see the results of an investment in ScienceLogic’s SL1 AIOps platform.
- Company
- Main Menu
- Company
Company
We’re on a mission to make your IT team’s lives easier and your customers happier.
- About Us
- Careers
- Newsroom
- Leadership
- Contact Us
- Virtual Event2024 Innovators Awards SpotlightRegister Now
Save your seat for our upcoming PowerHour session on November 20th.
News Roundup, May 20: What’s Happening in AIOps, ITOps, and IT Monitoring
On this day in 1927 at 7:40 AM, Charles Lindbergh took off from New York to cross the Atlantic for Paris, aboard the Spirit of St Louis. In honor of Charles and the first non-stop flight across the pond, see how your organization can reach new heights by reading the latest in AIOps, ITOps, and IT infrastructure monitoring.
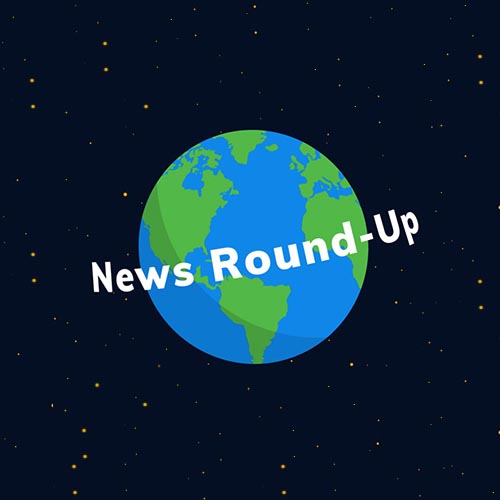
1. Here’s Forrester’s take on the differences between monitoring, observability, and AIOps.
An article in Forrester discusses the differences among monitoring, observability, and AIOps. When you think about monitoring, observability, and AIOps, ask yourself: How do veterinarians, physicians, and biomechanical engineers go about their jobs and work with their patients?
When you bring your new puppy to the vet, your pet can’t answer the questions. It simply behaves in its own way, and the vet must watch and infer what it is that might be wrong based on little or no direct patient feedback. The same holds true for a server or application that is being monitored. Moving from a veterinarian to a physician is not about the provider but about the patient. The physician can get actual feedback when treating a human patient. They can ask the patient what hurts, what’s worked in the past, and how they tolerate certain treatments.
Biomechanical engineers design systems that are partly mechanical and partly software for the betterment of human life. These biomechanical solutions consume real-time human metrics and provide capabilities needed at that moment in time to ensure the systems stay running and provide the best possible experience. They build upon monitoring and observation skills and then add advanced techniques to enhance what is possible, very similar to AIOps.
In the IT space, there is a natural progression from monitoring through observability and on to AIOps. These are not mutually exclusive needs but supportive and additive to the prior one. Organizations have and will always utilize all three approaches in their IT environments depending on their goals and objectives.
2. What’s the difference in the roles of DevOps, PrivacyOps, and AIOps in organizations today?
CPO Magazine compares DevOps, AIOps and PrivacyOps and assesses their roles in organizations.
Increased importance of automation, data protection statutes, and corresponding corporate practices has put DevOps, PrivacyOps, and AIOps center stage amongst organizations’ strategic goals.
DevOps are a set of practices that combine the functions of software development (Dev) with IT operations (Ops). DevOps have become a cornerstone of any organization’s ability to develop software more efficiently in the past couple of years. Rather than working in their individual silos, business requirements, coding, quality assurance, networking, and database teams work simultaneously on a project. As a result, communication between teams is far more proactive, ambiguities are resolved early, and feedback.
AIOps is the amalgamation of DevOps with artificial intelligence and machine learning. Using the latest AI and machine learning tools, an organization can gather, categorize, analyze, and visualize data on an unprecedented scale. This can prove immensely helpful to DevOps teams in making proactive changes in their products and services while providing actionable insights to aid future improvements. AIOps can increase any organization’s ability to have an automated plan in place that can map out all possible results of a particular approach.
PrivacyOps is the need of the hour for almost every organization dealing with tranches of user data. Like DevOps, PrivacyOps’ primary function is to increase the collaborative functions between the IT department and legal team to ensure an organization’s products and services are fully compliant with various data protection laws globally. The better an organization’s workforce adapts to the PrivacyOps framework, the better an organization’s ability to comply with data protection regulations.
3. What’s the long play for AIOps in enterprise data management?
This blog by Silicon Angle explores the usefulness of AIOps in enterprise data management.
There’s something of a gold rush toward automation through machine learning and AI as organizations grasp its multiple benefits. This automation should be embedded into every area of a solutions organization’s operations.
An unprecedented number of business processes are moving to the cloud from on-prem setups, and a new paradigm is emerging, dubbed “cloud-scale. The contemporary “lift-and-shift” movements are now making their way into IT operations, customer service, ticketing and even record management, and long may it continue. On the buying side of things, spending trends have also changed positively toward favoring solutions startups.
Today, most companies are already spending 20, 30% with startups. And it can double in the next four or five years. This increased spending is being driven by fresh-thinking startups developing new standards in security and cloud app development. The training of AI and machine learning models toward stronger predictions and autonomy would be key to sustaining this growth.
4. Data lakes are massively scalable storage units for big data analytics.
This article from Infoworld dives into data lakes, including what they are, how they’re used, and how to ensure your data lake does not become a data swamp.
Data lakes have evolved since then, and now compete with data warehouses for a share of big data storage and analytics. Various tools and products support faster SQL querying in data lakes, and all three major cloud providers offer data lake storage and analytics. There’s even the new data lake house concept, which combines governance, security, and analytics with affordable storage.
The question isn’t whether you need a data lake or a data warehouse; you need both, but for different purposes. These are the areas where major differences between data lakes and data warehouses occur:
- Data sources;
- Schema strategy;
- Storage infrastructure;
- Raw vs curated data;;and
- Type of analytics.