- Why ScienceLogic
- Main Menu
- Why ScienceLogic
Why ScienceLogic
See why our AI Platform fuels innovation for top-tier organizations.
- Why ScienceLogic
- Customer Enablement
- Trust Center
- Technology Partners
- Pricing
- Contact Us
- Product ToursSee ScienceLogic in actionTake a Tour
Experience the platform and use cases first-hand.
- Platform
- Main Menu
- Platform
Platform
Simplified. Modular-based. Efficient. AI-Enabled.
- Platform Modules
- Core Technologies
- Platform Overview
- Virtual ExperienceSkylar AI RoadmapView Now
Learn about our game-changing AI innovations! Join this virtual experience with our CEO, Dave Link and our Chief Product Officer, Mike Nappi.
- Solutions
- Main Menu
- Solutions
Solutions
From automating workflows to reducing MTTR, there's a solution for your use case.
- By Industry
- By Use Case
- By Initiative
- Explore All Solutions
- Survey ResultsThe Future of AI in IT OperationsGet the Results
What’s holding organizations back from implementing automation and AI in their IT operations?
- Learn
- Main Menu
- Learn
Learn
Catalyze and automate essential operations throughout the organization with these insights.
- Blog
- Community
- Resources
- Events
- Podcasts
- Platform Tours
- Customer Success Stories
- Training & Certification
- Explore All Resources
- 157% Return on InvestmentForrester TEI ReportRead the Report
Forrester examined four enterprises running large, complex IT estates to see the results of an investment in ScienceLogic’s SL1 AIOps platform.
- Company
- Main Menu
- Company
Company
We’re on a mission to make your IT team’s lives easier and your customers happier.
- About Us
- Careers
- Newsroom
- Leadership
- Contact Us
- Congratulations2024 Innovators AwardsView the Winners
See how this year’s winners have demonstrated exceptional creativity and set new standards in leveraging the ScienceLogic AI Platform to solve complex IT Ops challenges.
Three Use Cases for Implementing Machine Learning
Machine learning is compelling businesses to innovate in new ways. Here are three use cases that illustrate why machine learning is worth your investment.
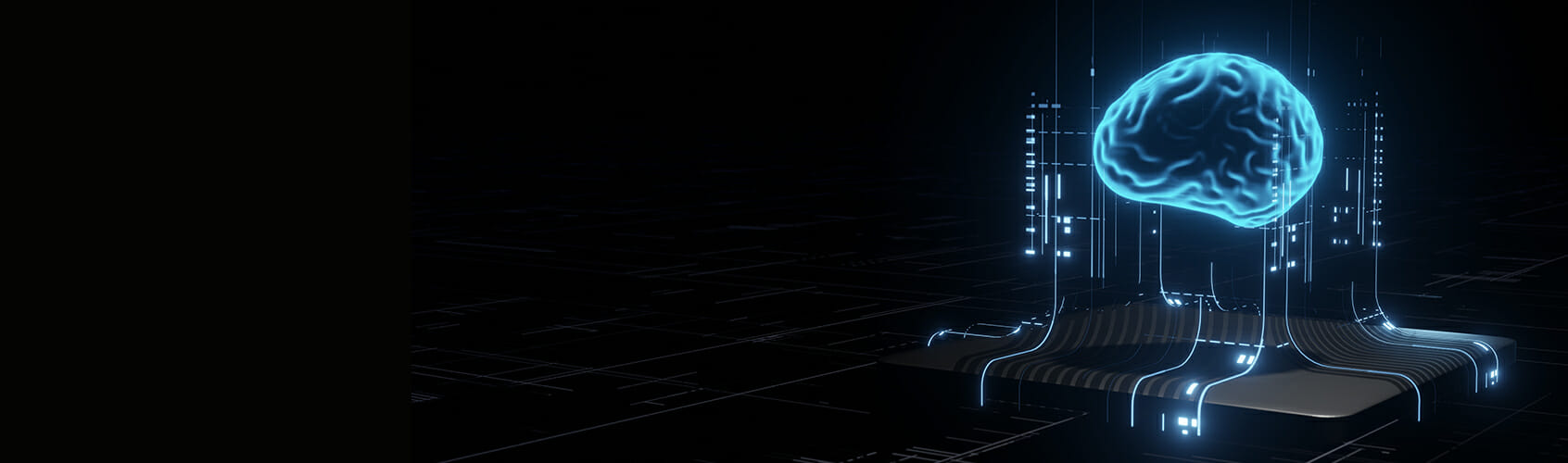
Advanced machine learning makes it possible to use data produced and collected from a wide variety of end point devices—from traditional IT infrastructure to IoT sensors—to manage operations in ways that were not possible just a few years ago. This has created new opportunities for cloud services providers and managed services providers to innovate as well, creating new service portfolios that support emerging business models.
All this innovation requires an investment in monitoring and management technologies that can apply machine learning to drive predictive operations that advise you on what actions you should take based on both real-time and learned behavior. Operational analytics based on machine learning aren’t tied to the application of rigid rules that are often irrelevant in a dynamic, fast-changing environment. Instead, they learn and adapt, making recommendations based on the current state of systems and service health as they both change moment to moment.
That is vital to keeping pace with the demands of today’s service environments. Cloud and hybrid networks, software defined storage and applications, and sprawling IoT device deployments are being used in highly specialized ways. When evaluating the changing needs of different customers and the associated demands each may have on a monitoring and management platform, here are three use case categories to consider.
Use Case 1: Predicting Storage Performance and Capacity
The era of purchasing excess storage to ensure you’ll have enough when the need arises, or to accommodate possible long-term growth is over. That approach is expensive, inefficient, and may not address performance issues that happen when demand spikes or issues occur. With an IT operations platform powered by machine learning, you can monitor conditions that require the acquisition and allocation of storage capacity based on what’s happening now and what’s been happening over time, and forecasts the needs to come. You can also respond—automatically—to events that can help forestall catastrophic failures or performance degradation. By making intelligent decisions based on an accurate, contextual reckoning of the state of operations across business services, you can ensure the most efficient allocation of capacity, the most effective performance, and minimal downtime.
Use Case 2: Predicting CPU Performance and Capacity
Effective management of CPU resources in support of business services means asking a number of questions:
- Do I have enough performance and CPU capacity, and is there capacity available for me to shift workloads around?
- How many gigahertz are available across the entire ecosystem at any moment to give to an application, and what type of CPU can I allocate?
- How much standby CPU inventory (not already online) is available for me to use?
Answering these questions involves a number of layers of consideration, including operating system, VMWare layer, physical host/blade usage (including placement of blades in different chassis), and cluster type. Once you’ve taken a complete inventory you need to understand the multilayered service relationships and then detect when anything changes.
Doing this manually, or with traditional IT operations platforms, is impossible. With machine learning, however, you can account for all these variables in real time, predict needs, and dynamically adjust service operations when resources are added, removed, or otherwise changed. This enables you to buy additional capacity in anticipation of forecasted need or adjust down if capacity reduces.
(It’s worth noting that, while VMware can claim to provide some predictive insights, VMware has zero context of the application view or of anything operating in any layer other than VMware. It also doesn’t have a concept of other capacity that may be available.)
Use Case 3: Predicting an AWS Outage
When you provide (or rely on) outsourced infrastructure, it is vital to know what’s happening in order to avoid costly downtime. Gartner estimates the average cost of downtime at roughly $300,000 per hour. For high-volume operations, the costs can be much higher. In May of 2017, the CEO of British Airways explained how one service failure stranded tens of thousands of their passengers, costing the company $102.19 million.
The application of machine learning to avoid catastrophic failures demands complete, accurate, and real-time data—analyzed in the context of the entire IT environment supporting business services—to predict events, and to automate the steps required to avoid or minimize downtime.
We have a deep experience working with MSPs and cloud service providers using the machine learning behind the ScienceLogic SL1 platform to help solve a variety of complex challenges and accommodate the shift from reactive to predictive IT management—enabling service automation so that you can focus your resources on delivering top quality service and building your business. Using SL1 as the conduit for collecting and preparing a clean data lake, you can apply intelligent analysis to support decision making and drive actions while continuously retraining and enhancing your operation models based on new data and response to anomalies.
If you want to learn more about how ScienceLogic can help implement machine learning for a company like yours, register for this webinar.