- Why ScienceLogic
- Main Menu
- Why ScienceLogic
Why ScienceLogic
See why our AI Platform fuels innovation for top-tier organizations.
- Why ScienceLogic
- Customer Enablement
- Trust Center
- Technology Partners
- Pricing
- Contact Us
- Product ToursSee ScienceLogic in actionTake a Tour
Experience the platform and use cases first-hand.
- Platform
- Main Menu
- Platform
Platform
Simplified. Modular-based. Efficient. AI-Enabled.
- Platform Modules
- Core Technologies
- Platform Overview
- WebinarCompliance Meets Security—No Drama RequiredRegister Now
Join our live webinar to explore how integrating compliance into your network security keeps your policies airtight and your infrastructure secure.
March 27, 2025 | 11am ET
- Solutions
- Main Menu
- Solutions
Solutions
From automating workflows to reducing MTTR, there's a solution for your use case.
- By Industry
- By Use Case
- By Initiative
- Explore All Solutions
- Survey ResultsThe Future of AI in IT OperationsGet the Results
What’s holding organizations back from implementing automation and AI in their IT operations?
- Learn
- Main Menu
- Learn
Learn
Catalyze and automate essential operations throughout the organization with these insights.
- Blog
- Community
- Resources
- Events
- Podcasts
- Platform Tours
- Customer Success Stories
- Training & Certification
- Explore All Resources
- 157% Return on InvestmentForrester TEI ReportRead the Report
Forrester examined four enterprises running large, complex IT estates to see the results of an investment in ScienceLogic’s SL1 AIOps platform.
- Company
- Main Menu
- Company
Company
We’re on a mission to make your IT team’s lives easier and your customers happier.
- About Us
- Careers
- Newsroom
- Leadership
- Contact Us
- Congratulations2024 Innovators AwardsView the Winners
See how this year’s winners have demonstrated exceptional creativity and set new standards in leveraging the ScienceLogic AI Platform to solve complex IT Ops challenges.
MTTR vs. MTTA vs. MTBF: A Complete Set of Common Incident Management Metrics
There are a common set of key performance indicators for incident management, such as MTTR and MTTA. What do these metrics mean, and why are they important?
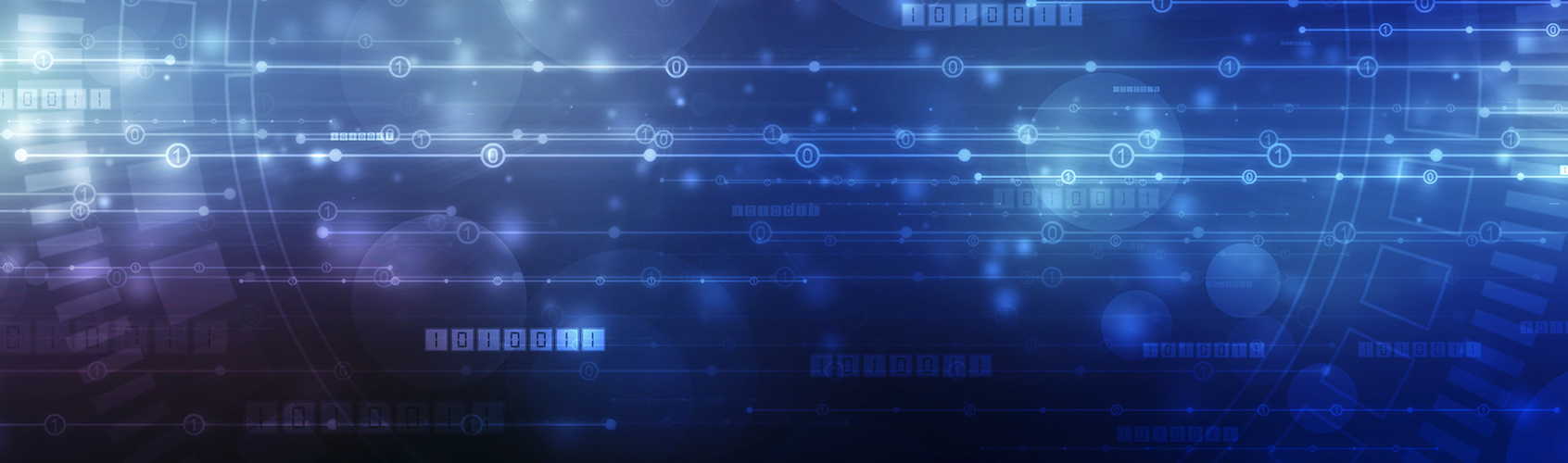
List of Incident Management Metrics
These metrics are crucial to understanding, diagnosing, and fixing the issues that prevent your IT infrastructure from operating at peak efficiency.
These metrics include:
- Mean Time to Detection (MTTD) is the time it takes for an issue to be identified as one requiring attention;
- Mean Time to Acknowledge (MTTA) is the average time required for an alert to result in the initiation of action, usually the issuing of a service ticket, on the part of the IT operations team;
- Mean Time to Respond (MTTR) is the average time it needs to begin the work associated with a service ticket;
- Mean Time to Repair (MTTR) is the time it takes from the point of detection until the system is fixed;
- Mean Time to Resolve (MTTR) is the time needed to move from the point of detection until the system is fixed and tested to ensure the associated system is working properly; and
- Mean Time to Recovery (MTTR) is the time required to go from the point of detection to when the associated system is fully operational.
There are two other Mean Time KPIs that are used to determine how effective you are at operating and supporting your digital services in regards to MTTR. And while you want your MTTx numbers to be small, you want your MTB numbers to be large.
- Mean Time Between Failure (MTBF) is the time between incidents of a particular device or application
- Mean Time Between System Incidents (MTBSI) is the average time between failures of a system or service rather than the failure of any single component of the system or service
Unfortunately, over time some of these metrics have been overlooked, neglected, and even ignored. This can result in inconsistent and deceptive performance measurements that are reflected in the substandard health, availability, and reliability of the services and applications you rely on to achieve your mission objectives. But it doesn’t have to be this way.
Overwhelmed by Complexity
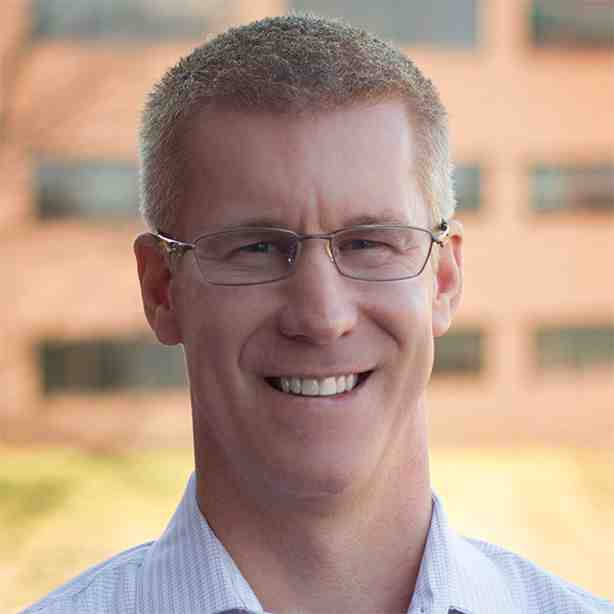
In an ironic twist, the above situation came about due to the evolution of the enterprises they were meant to help with MTTR. As IT estates grew in size and complexity, it became more difficult to keep up with the volume of events – and more recently, anomalies – generated. To deal with the growth of issues, tools multiplied, and staffs grew. But eventually, an enterprise reaches a point of diminishing returns or runs up against budget constraints.
That’s when your ITOM staff starts looking for other ways to adapt while offering the best possible service level. They do the best they can to prioritize and fix the biggest issues as quickly as they can, and they develop workarounds for the rest. That includes the convenient omission of some of those pesky MTTx KPIs.
But there’s a reason those KPIs exist. There’s a measured process for addressing incidents, and how you measure the speed and efficacy of your incident management process matters. If you don’t measure each step consistently every time, you can’t determine whether you’ve actually fixed the problem or if you’ve merely suppressed the symptom. That’s why there is a complete set of common incident management metrics, and why they are in their order: detect, acknowledge, respond, repair, resolve, and recover.
Mean Time to Repair (MTTR) Formula
MTTR is discovered through a mathematical equation where the total amount of corrective failures is divided by the entire number of corrective actions and failures during a certain time period. This measurement includes both actual repair time as well as the time required to properly test the system to make sure the fault is fully gone. The time measured for MTTR begins when the problem has been reported and it does not pause until everything has been fixed, tested, and reported to be complete.
An example of how MTTR is measured is when a system failure is discovered on a Monday. There were 12 outages, and the systems were being fixed for six hours on that day. So that is six hours multiplied by 60 minutes, which adds up to 360 minutes of repair time. That is divided by the number of outages that took place, that in this case was 12, so the MTTR is 30 minutes.
What is considered good MTTR?
Many factors need to be considered when defining “good MTTR.” But a good rule of thumb for time to repair or recovery is under five hours.
Incident Metrics Step-by-Step
When an incident is identified, it triggers an alert. That’s when the clock should start ticking. In my experience, 90% of companies don’t start measuring for any MTTx results until a ticket is created. When you skip steps in the process, however, you manipulate the results of MTTR, and when that happens, and the incident goes away, or the supporting data necessary for performing triage and diagnosis is not captured, potential problems are dismissed as “noise” in the system.
For example, if an event occurs and is identified at 12:00 pm MTTD, but the incident isn’t opened MTTA until 1:00 pm and that is when you begin your measurement, you’ve not only artificially cut an hour from your MTTR calculation, but you’ve also lost the data associated with the event that could have provided key insights for triage and, ultimately, a diagnosis that allows you to repair the device or application, and to find and fix the underlying problem. You came away from the incident with a good MTTR, but you have also doomed yourself to a recurring issue and, notably, poor metrics for MTBF and also MTSBI (if you’re even keeping track of those).
Don’t Take Shortcuts
These measurements were created because they are essential to determining the state of a repair and creating a set of best practices to track. When you follow the proper process and don’t rely on shortcuts or workarounds, you end up with a better result in the long run. Because some repairs require specific processes—such as a full system restart—to complete, skipping or ignoring steps might even result in missing a simple fix that prevents a complete recovery. When you see the same incidents over and over again, you may have a Mean Time to Repair of 30 minutes, but no Mean Time to Respond because the machine or application is still in an error state.
These time-wasting exercises bedevil organizations that fail to track and record all of the MTTx KPIs. If you aren’t tracking MTBF, (and few organizations do) and rely on workarounds and shortcuts to resolve incidents, you will fail to recognize weaknesses in the system or capture the data necessary to fix the problem. Over time, and despite the likelihood of serious system failures and even SLA violations, it will be difficult to isolate the reasons why, because your MTTR figures seem strong.
Why does that happen? Workarounds are the bane of IT operations. They hide evidence of poor performance while providing just enough plausible deniability of an actual problem to allow things to continue uncorrected. Every enterprise uses them to some degree, most often by necessity. You do what you can with what you have to keep the operation running, after all. Fortunately, there is a better way.
Machine Speed and AIOps
When configured correctly, a machine will respond to signals that fall outside of acceptable thresholds—or act ‘weird’ when evaluated against normal patterns of behavior. It doesn’t get fatigued or frustrated by the same incident recurring, no matter how quickly after a resolution, or how often. A machine has its instructions and tells you when something is not normal. It has no emotional stake in that decision and has no concern about its next performance review. But the more these problems affect human beings, the more likely those concerns are to affect the process, and operational processes not built on these metrics are doomed to fail. But so are ITOM teams that are expected to follow a process that hews to these metrics, but without the tools capable of operating with the speed and accuracy required by today’s modern IT estates.
That is where AIOps comes to play. We can revive the full set of KPIs and achieve better ITOM results when we rely on machines with the speed and intelligence to keep pace with the volume, variety, and velocity of incidents. An AIOps platform, like ScienceLogic SL1, is purpose-built for the task of monitoring the totality of today’s complex IT estates and capturing all the relevant data necessary to understand the health, availability, and reliability of all systems and services. You can measure all your KPIs and use them as a measurement to keep your services, infrastructure, and applications running at their best. What’s more, with an AIOps platform like SL1, you can build toward using the inherent intelligence and speed of the platform to build toward the automation of incident response. All those nagging signals that you’ve been treating as noise in the system can be addressed quickly and completely, and you can use the associated data to better understand the big picture of your system’s efficient operations.
Is your organization among those struggling to keep up with the volume, variety, and velocity of incidents and their associated data? Is your organization still using legacy tools and brute force to monitor and manage an increasingly complex IT estate? If so, it’s time to modernize and move up to an AIOps platform.
See how Capgemini Reduced MTTR
Forrester Consulting examines how Capgemini IT completely transformed the firm’s global network operations center to reduce MTTR and operational costs.