- Why ScienceLogic
- Main Menu
- Why ScienceLogic
Why ScienceLogic
See why our AI Platform fuels innovation for top-tier organizations.
- Why ScienceLogic
- Customer Enablement
- Trust Center
- Technology Partners
- Pricing
- Contact Us
- Product ToursSee ScienceLogic in actionTake a Tour
Experience the platform and use cases first-hand.
- Platform
- Main Menu
- Platform
Platform
Simplified. Modular-based. Efficient. AI-Enabled.
- Platform Modules
- Core Technologies
- Platform Overview
- DemoExperience the Power of ScienceLogicRegister Now
See how today’s technology leaders are reducing costs, streamlining IT operations, and improving the customer experience.
- Solutions
- Main Menu
- Solutions
Solutions
From automating workflows to reducing MTTR, there's a solution for your use case.
- By Industry
- By Use Case
- By Initiative
- Explore All Solutions
- Survey ResultsThe Future of AI in IT OperationsGet the Results
What’s holding organizations back from implementing automation and AI in their IT operations?
- Learn
- Main Menu
- Learn
Learn
Catalyze and automate essential operations throughout the organization with these insights.
- Blog
- Community
- Resources
- Events
- Podcasts
- Platform Tours
- Customer Success Stories
- Training & Certification
- Explore All Resources
- Upcoming WebinarStack the Deck in Your Favor: Automation for MSP ExcellenceRegister Today
From predictive analytics to auto-remediation, learn how to turn a full house of IT problems into a royal flush of solutions in this live webinar.
April 30, 2025
- Company
- Main Menu
- Company
Company
We’re on a mission to make your IT team’s lives easier and your customers happier.
- About Us
- Careers
- Newsroom
- Leadership
- Contact Us
- Congratulations2024 Innovators AwardsView the Winners
See how this year’s winners have demonstrated exceptional creativity and set new standards in leveraging the ScienceLogic AI Platform to solve complex IT Ops challenges.
AIOps Part 2: What Flavor of Machine Learning is Right for You?
We covered the broad strokes of artificial intelligence (AI), machine learning (ML), and the differences between the two in our last blog. Now let’s delve much deeper into ML.
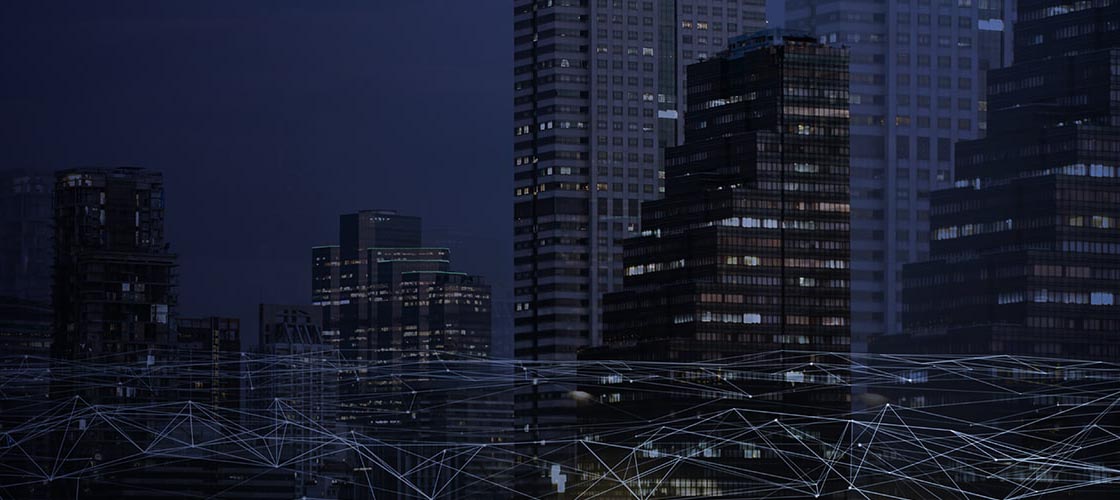
There are often multiple ways to solve the same problem. With ML, it’s important to recognize that there are three primary flavors of ML you can choose from to solve IT problems:
- Supervised learning, where the algorithm is taught by example.
- Unsupervised learning, where the algorithm attempts to learn and extract patterns on its own.
- Reinforcement learning, which, unlike supervised and unsupervised learning, starts only with a mathematically defined objective, and uses data inputs to find the best way to achieve or optimize that objective.
Each flavor offers a wide variety of algorithms to choose from. And you may choose to apply one or more of these types of ML to understand your IT environment, reach conclusions, make decisions, and address problems that impact your customer and employee experience more efficiently. But which approach will yield the best results for you?
This is where the how of implementing ML that we discussed in the previous article becomes critically important. Defining the problem to solve is the first step. The next step is determining which type of ML to use: for example, is it only an unsupervised learning problem, or can it and should it be reframed as a supervised learning problem? And depending upon which type you pick, which algorithm do you use within that category? And then which values do you choose to tune the parameters associated with the algorithm? Each answered question introduces yet another set of options, making it abundantly clear that having a human make all these choices for each individual metric simply will not scale without an army of data scientists. Organizations require an AIOps solution that automatically makes these choices for them. To understand this more, let’s take a look at a specific problem: Anomaly detection.
Using ML to Detect Anomalies
Automatic detection of anomalous behavior that is uncharacteristic of a given signal (anomaly detection) is one of the most common IT problems addressed with ML today. You can frame this problem as supervised or unsupervised, with each approach offering a range of algorithmic options. Given this variety of choices, how do you assemble the essential ingredients of machine learning to empower you to detect anomalies in signals at a massive scale?
Before we fully answer that question, it’s important to understand that there is no single template for what constitutes normal behavior in any IT environment. The IT environments, digital services, and goals of each organization will differ widely, and so the optimal modeling approach may vary, and even change over time.
If your IT organization’s goal is to proactively detect anomalies across your IT environment, understand their impact on the business, and identify when and where there are problems to be solved, it’s crucial to invest in an AIOps solution that automatically selects the best-performing anomaly detection algorithm for each metric and node. And that algorithm must understand the nuances affecting the signals generated by each node and be capable of recognizing when an anomaly is an appropriate response to the environment’s current state, or when an anomaly is a symptom of a potential problem. And this is where ScienceLogic SL1 shines.
Using SL1’s Model Selector to Find the Best Algorithmic Model
The ScienceLogic SL1 Model Selector automatically chooses the best algorithmic approach—or approaches—aligned to your specific environment. Without Model Selector, your IT operations team would need a contingent of data scientists constantly monitoring your AIOps platform, adjusting every model, for every change in the environment. Every highly skilled data scientist knows, at any substantial scale, this is simply not practical. Conditions change too often for humans to keep up in the most basic enterprise conditions, and the chance for poor model fit would be too high, which introduces significant risk to the business.
Instead, the SL1 Model Selector ingests historical performance data, models the outcomes of each approach based on that data, and determines which algorithm is best suited for modeling the metric at hand. As more data is generated and ingested by SL1, and your operational goals evolve, SL1 Model Selector will self-adjust to ensure the right model is applied.
When Model Selector is used, SL1 detects and classifies out-of-scope observations for the nodes being monitored, correlates signals against historical data and policy, and determines if the anomaly is problematic or not. Over time, the optimal solution and recommended course of action will change as SL1’s algorithms are enriched with more data making your operation more efficient and ensuring optimal performance and reliability. And, as new algorithms are developed and made available, they will be seamlessly integrated into the array of options from which Model Selector can choose.
While many organizations are investing in ML-based anomaly detection, there is a potential downside to generating anomalies in addition to the typical alerts produced by your monitoring tools. Like traditional alerts, anomalies by themselves are not necessarily bad. You would not want humans to react every time something “weird” happens. Sometimes they are crucial evidence but other times they are transient blips that don’t impact the broader service.
Using SL1 Behavioral Correlation to Accelerate Root-Cause Analysis
Monitoring for anomalous behavior at the scale that SL1 Model Selector permits has the potential to produce a massive influx of new information regarding the behavior of your system. The overwhelming volume of anomalies compounds the number of incidents and events operators need to sift through. To address this, organizations require more than just a dedicated user interface for viewing a long list of anomalous behavior. They require an AIOps solution that analyzes and correlates anomalies and events within a service context to reduce the noise, accelerate root cause analysis, and recommend a set of triage/remediation actions. This is where Behavioral Correlation comes in to save the day.
To better understand why ScienceLogic SL1 is a leader in AIOps, check out the EMA Report, and keep your eyes peeled for the third and final blog in our series when we examine how ML supports behavioral correlation.