- Why ScienceLogic
- Main Menu
- Why ScienceLogic
Why ScienceLogic
See why our AI Platform fuels innovation for top-tier organizations.
- Why ScienceLogic
- Customer Enablement
- Trust Center
- Technology Partners
- Pricing
- Contact Us
- Product ToursSee ScienceLogic in actionTake a Tour
Experience the platform and use cases first-hand.
- Platform
- Main Menu
- Platform
Platform
Simplified. Modular-based. Efficient. AI-Enabled.
- Platform Modules
- Core Technologies
- Platform Overview
- Virtual ExperienceSkylar AI RoadmapView Now
Learn about our game-changing AI innovations! Join this virtual experience with our CEO, Dave Link and our Chief Product Officer, Mike Nappi.
- Solutions
- Main Menu
- Solutions
Solutions
From automating workflows to reducing MTTR, there's a solution for your use case.
- By Industry
- By Use Case
- By Initiative
- Explore All Solutions
- Survey ResultsThe Future of AI in IT OperationsGet the Results
What’s holding organizations back from implementing automation and AI in their IT operations?
- Learn
- Main Menu
- Learn
Learn
Catalyze and automate essential operations throughout the organization with these insights.
- Blog
- Community
- Resources
- Events
- Podcasts
- Platform Tours
- Customer Success Stories
- Training & Certification
- Explore All Resources
- 157% Return on InvestmentForrester TEI ReportRead the Report
Forrester examined four enterprises running large, complex IT estates to see the results of an investment in ScienceLogic’s SL1 AIOps platform.
- Company
- Main Menu
- Company
Company
We’re on a mission to make your IT team’s lives easier and your customers happier.
- About Us
- Careers
- Newsroom
- Leadership
- Contact Us
- Congratulations2024 Innovators AwardsView the Winners
See how this year’s winners have demonstrated exceptional creativity and set new standards in leveraging the ScienceLogic AI Platform to solve complex IT Ops challenges.
Why You Don’t Need a Data Scientist: Automatically Selecting the Right Anomaly Detection Model
What if you could ensure the right model is deployed for each device and metric you monitor—without hiring a data scientist or even writing one line of code?
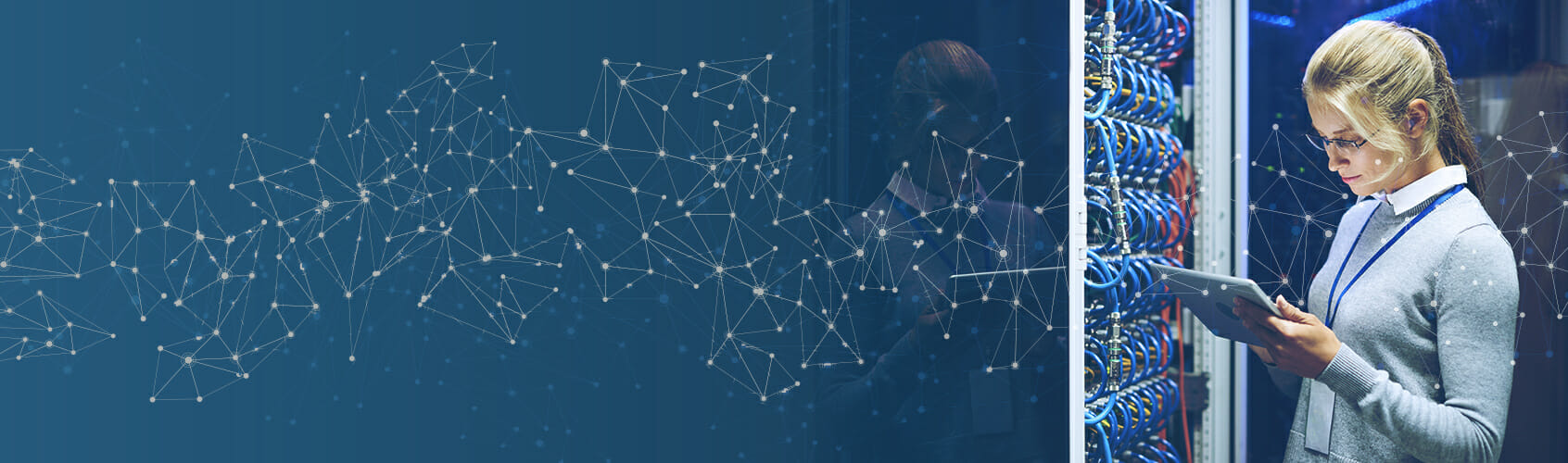
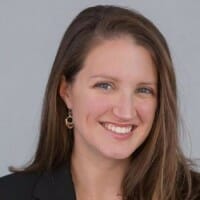
Anomaly detection is the process of discovering whether the behavior on your device is normal or not. Although anomalies are not always a sign of bad behavior, knowing which of your devices are behaving abnormally during an outage can be the difference between catching a problem before your customers are affected or after. For that reason, it’s essential to have the best-performing anomaly detection algorithm fit to each metric and device you select.
ScienceLogic’s anomaly detection approach does not provide for a single algorithm but instead allows the model to inform us which of the available algorithms best fits the data. A model then detects anomalies using a single permutation of the algorithm and hyperparameters that best fit the specific data from a single metric on a single device.
The model that fits the best will depend on a variety of factors specific to the selected data for each device you wish to monitor. Factors such as the polling frequency, behavior variation, behavior seasonality, and the data type will all influence the performance of anomaly detection for a given set of metric data.
If you plan to monitor multiple devices with various metrics in a traditional AI/ML approach, you would have to manually tune a specific model for each of them to get optimal results. This high-code, high-math version is time-consuming, and may even require the expertise of a data scientist.
What if you don’t have a data scientist available for this task?
The Machine Learning Team at ScienceLogic has created an automatic model selector (Autoselector) that can find the best-fit algorithm for each device and metric you enable—already tuned and ready to detect anomalies in your data.
The main design goal behind Autoselector is to drastically simplify the process of enabling machine learning on a single device–or a large swath of devices–you wish to monitor. The design is consistent with the process in SL1 for a user to set preferences while creating any other monitoring policy. A current SL1 user now has a tool to monitor devices and solve problems more quickly without writing a single line of code.
The Autoselector works by ingesting historical data from a metric on one device, which is then analyzed and scored in various arrangements of algorithms and hyperparameters until an ultimate “winner” is selected. My team and I like to think of it as an algorithm fight club.
How does an algorithm win?
First, the Autoselector takes in historical data from a metric and runs a check for sufficient data and whether the data type is available for analysis. Once it has determined enough historical data exists, it runs through a process called the Monte Carlo method.
Using this Monte Carlo method, the Autoselector creates a set of scenarios in which the algorithms and the hyperparameters for those algorithms are all randomized. The same dataset runs through every scenario, and a score produced for each one. The score is a reflection of how well anomalies can be distinguished from normal data, and the scenario with the highest score wins.
The Autoselector then stores the winning algorithm–pre-trained on the data that was initially ingested–in ScienceLogic’s proprietary model registry, where the pre-trained model will reside. This process is set into motion for each metric you select for anomaly detection, and it can be re-triggered at any time.
What happens when you turn Autoselector on?
With the best model selected and uploaded to the model registry, when a new time-series observation for the selected metric comes in, it will begin detecting new anomalies and periodically update its training based on new incoming data.
These anomalies will generate an alert in SL1. Each time the model classifies a new time-series observation on the device you are monitoring for anomalies, it will save that classification and send it to the SL1 user interface connected to that policy. There you will be able to click and see a graph that shows if that new datapoint is anomalous or not.
You can also create event policies and automation policies around anomaly alerts. Automation policies can take immediate action on anomalies, such as “send a notification,” or “perform some further diagnostics.” You can use the full power of SL1 event policies to capture that behavior (e.g., “notify when X number of anomalies happen during Y time”), which is extremely helpful, especially if anomalies for this metric correlate to some otherwise-difficult-to-detect behavior in the system.
Below is a visual of how the Autoselector and anomaly detection services interact with each other and the broader system in SL1:
SL1 empowers the user to find and view anomalies, so that they can find the source of a problem when it occurs more quickly. When an outage occurs, the user will be able to quickly identify all the devices that currently have anomalous behavior–narrowing the field for finding a root cause.
The Autoselector ensures that you have the right model deployed for each device and metric you monitor. And you don’t need to hire a data scientist or even write one line of code.
Want to learn more about ML-assisted automation? Watch this webinar >