- Platform
- Main Menu
- Platform
- Platform Overview
- SL1
- Skylar AI
- Skylar Advisor
- Skylar Analytics
- Skylar Automated RCA
- Restorepoint
- Hybrid Cloud Monitoring
- Multi-Cloud Monitoring
- Network Monitoring
- Integrations
- Trust Center
- Technology Partners
- Why ScienceLogic
- Compare
- Virtual KeynoteWatch the Video
Introducing Skylar AI: the suite of advanced AI capabilities that will redefine productivity, creativity, and decision-making across industries.
- Solutions
- Main Menu
- Solutions
- By Industry
- Solutions
- By Industry
- Enterprise IT Solutions
- Global System Integrators
- Service Providers
- Government & Public Sector
- Financial Services
- Channel Partners
- Learn More
We saw a better than 80% reduction in incident-related noise.
Download the Forrester Total Economic Impact™ which examined four enterprises with large, complex IT estates to measure the value and return on investment of ScienceLogic's AIOps Solution.
- By Solution
- Solutions
- By Solution
- AIOps Digital Transformation
- Business Service Management
- Tool Consolidation & Modernization
- IT Workflow Automation
- IT Infrastructure Monitoring
- Network Management
- Network Compliance
- Learn More
We saw a better than 80% reduction in incident-related noise.
Download the Forrester Total Economic Impact™ which examined four enterprises with large, complex IT estates to measure the value and return on investment of ScienceLogic's AIOps Solution.
- By Use Case
- Solutions
- By Use Case
- Accelerate Incident Response with Automated ITSM Workflows
- Automated Troubleshooting & Remediation
- Eliminate Visibility Gaps with Hybrid Cloud Monitoring
- Automate PCI DSS Compliance Checks for Network Devices
- Learn More
We saw a better than 80% reduction in incident-related noise.
Download the Forrester Total Economic Impact™ which examined four enterprises with large, complex IT estates to measure the value and return on investment of ScienceLogic's AIOps Solution.
- Customers
- Resources
- About
From Siloed IT to Coordinated IT: Navigating the First Steps Towards Autonomic IT
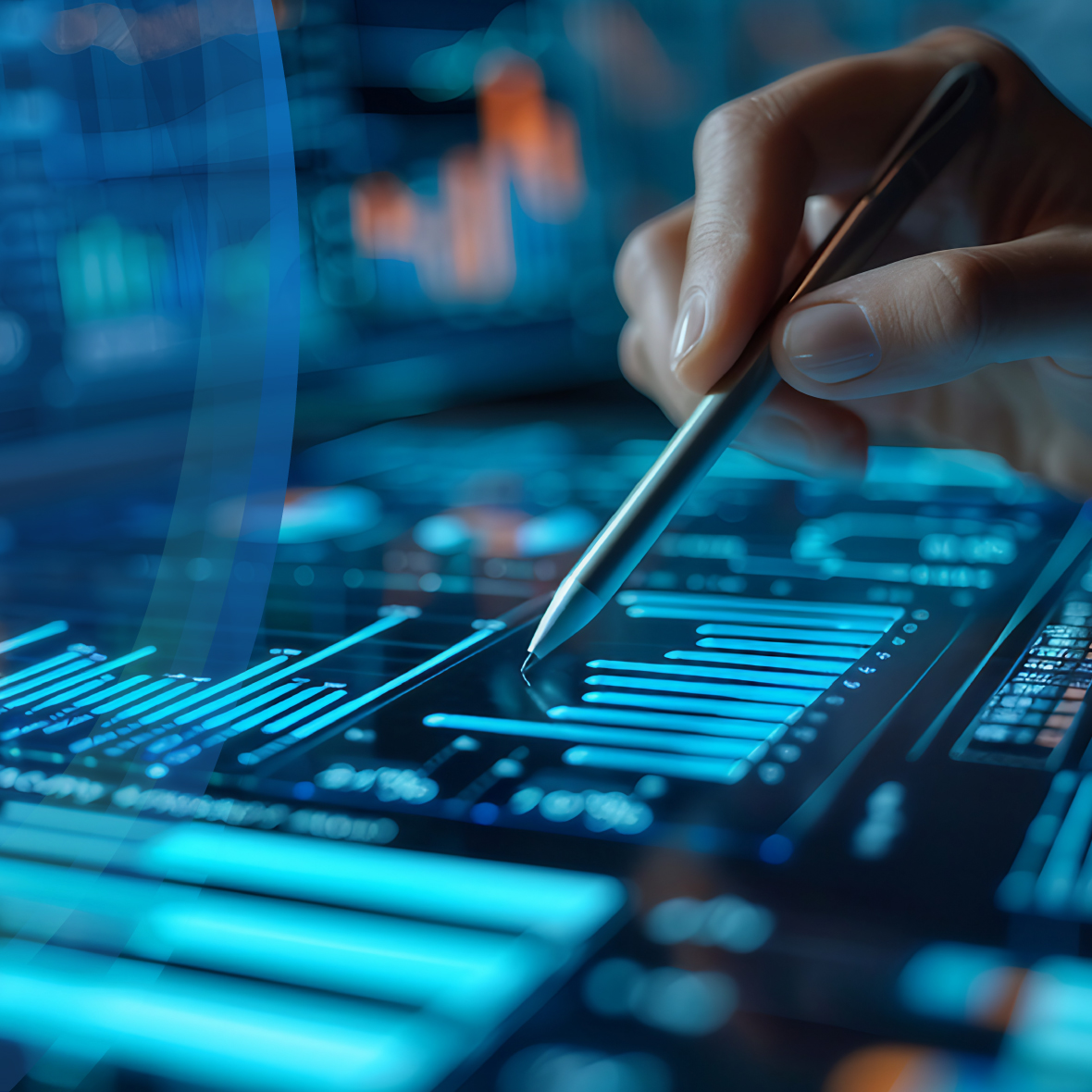
From Siloed IT to Coordinated IT: Navigating the First Steps Towards to Autonomic IT
Imagine a world where IT runs itself, monitoring and optimizing technology investments as it runs. Where IT operations are continuous: always available, always responsive, always seamless, always delivering what your organization – and your customers – need. This is Autonomic IT.
However, implementing Autonomic IT is not as simple as adding technology and flipping a switch. It involves a strategic five-phase journey to gradually transform traditional IT operations into a fully autonomous system.
These phases progress from highly-manual, human-powered operations carried out in silos and resulting in high costs, poor experience, and lost revenue. The journey culminates in the most advanced stage of Autonomic IT, where organizations realize a self-healing and self-optimizing IT infrastructure with highly efficient automated processes that minimize operational team dysfunction and free up time to generate new revenue opportunities.
Where Are You on Your Autonomic IT journey?
In reality, most organizations find themselves trapped at the lower levels of Autonomic IT maturity, where processes are often reactive and heavily reliant on manual, human-driven, and tool-intensive interventions. With too much time spent fighting fires and not enough time driving innovation, this reality poses significant obstacles to harnessing the full potential of AI and automation.
However, there is hope. By taking strategic steps, organizations can advance their Autonomic IT capabilities and progress toward higher maturity levels.
In this blog, the first in a series about journeying to Autonomic IT, we explore the characteristics of Phase 1 and outline the necessary steps to transition to Phase 2 and beyond.
Phase 1: Siloed IT
In today’s enterprise landscape, IT departments often find themselves trapped in a state of siloed operations and reactive troubleshooting. This situation is typically characterized by disparate tools, fragmented data, and isolated teams – collectively known as Siloed IT.
In this phase, different teams – including network engineers, Windows engineers, Linux engineers, cloud services engineers, AI engineers, and so on – each utilize domain or technology-specific monitoring tools. These tools, whether well-known brands or custom solutions, operate in isolation, albeit with varying degrees of automation.
The result is a chaotic environment or “data swamp” where data is trapped within silos, making it impossible to gain a unified view of the IT landscape and system-wide health. This fragmentation leads to several challenges:
- Delayed incident response due to the time required to gather data from multiple sources.
- Difficulty identifying root causes due to a lack of a holistic perspective and fragmented insights.
- Inefficient resource allocation as teams work in isolation, reactively troubleshooting in their disparate toolsets.
- Missed opportunities for cross-functional collaboration and knowledge sharing.
Against this backdrop, CIOs are seeking an “easy button” or quick solution to their challenges. For many, that solution lies in the power of AI.
AI in operations (AIOps) aims to liberate IT teams from the manual grunt work of managing complex IT infrastructures and enhance service quality through intelligent, data-driven insights and automation. But there’s a catch.
For AI to operate effectively, it needs machine-learned, structured data. But in siloed IT environments, data from various tools is disparate and unstructured. This scattered data reflects only random, isolated IT events, making it hard for AI to establish the necessary context to understand the significance and impact of individual events, diagnose root cause, and generate accurate, actionable insights that IT teams can act on.
As a result, engineers remain stuck in reactive mode, responding to incidents as they arise (at a rate of hundreds if not thousands each day) navigating a data swamp, unsure what to look for, and only fixing issues within their specific domain.
This lack of coordination means that problems are addressed in isolation rather than managed holistically – a must in today’s connected IT ecosystems where all levels of the IT infrastructure play a role in supporting business services. Moreover, IT teams miss the opportunity to work proactively and collaboratively leveraging intelligent, shared data-driven insights to optimize IT operations and prevent future incidents. Instead, they stick to their tried and tested tools, practices, and processes, reluctant to adapt and change.
If this sounds like your organization – bogged down with siloed tools, a data swamp, and reactive troubleshooting – let’s explore how you can move towards the next phase, where tools are consolidated, better insights are gleaned, and costs are reduced.
Phase 2: Coordinated IT
The shift from Siloed IT to Coordinated IT involves breaking down those data silos, consolidating tools, and integrating processes across teams into a unified framework for powerful visibility across the IT estate.
The first step in this process is to converge data from previously isolated systems into a contextualized data lake for more meaningful analysis, coordination, and enhanced decision-making.
This is where the ScienceLogic AI platform comes into play. ScienceLogic centralizes and normalizes data in a data lake, cuts through the noise created by multi-dimensional data flows, and then applies context to make it more meaningful and actionable.
If you think about it, operational patterns leave “breadcrumbs.” ScienceLogic connects the dots between them, discovering patterns and capturing how elements in an IT ecosystem work together. Using ScienceLogic’s data-aware approach, engineers can quickly determine the incident’s business service impacts and collaborate to identify where the root cause truly lies – network, database, cloud, and so on – for faster, more proactive resolution.
With this coordinated insight, they can lay the groundwork for the future phases of Autonomic IT and apply machine learning and analytics to craft IT workflow automations.
Instead of merely fixing problems, engineers can begin teaching the platform to resolve routine issues automatically by executing all necessary triage steps. Over time, guided by humans, the system will learn to perform these tasks autonomously, freeing engineers from the manually intensive task of sifting through logs and an abundance of data to find and triage incidents so they can focus on strategic IT optimization initiatives and propel the business forward.
That’s why Phase 2 is so crucial. To effectively manage data volume and velocity and unlock the full potential of AI, machine learning, and advanced Autonomic IT, you must first move beyond siloed IT practices and develop a cohesive, coordinated IT process.
Coming Soon: Advancing to Phase 3: Machine-Assisted IT
In the next blog in this series, we’ll explore how to progress your Autonomic IT journey from Phase 2 to Phase 3. A stage characterized by machine-assisted IT, where IT systems are not just responding to incidents but actively predicting and preventing issues.
Learn more about the Autonomic IT journey or contact our Advisory Services to help you determine your Autonomic maturity.