- Why ScienceLogic
- Main Menu
- Why ScienceLogic
Why ScienceLogic
See why our AI Platform fuels innovation for top-tier organizations.
- Why ScienceLogic
- Customer Enablement
- Trust Center
- Technology Partners
- Pricing
- Contact Us
- Product ToursSee ScienceLogic in actionTake a Tour
Experience the platform and use cases first-hand.
- Platform
- Main Menu
- Platform
Platform
Simplified. Modular-based. Efficient. AI-Enabled.
- Platform Modules
- Core Technologies
- Platform Overview
- Virtual ExperienceSkylar AI RoadmapView Now
Learn about our game-changing AI innovations! Join this virtual experience with our CEO, Dave Link and our Chief Product Officer, Mike Nappi.
- Solutions
- Main Menu
- Solutions
Solutions
From automating workflows to reducing MTTR, there's a solution for your use case.
- By Industry
- By Use Case
- By Initiative
- Explore All Solutions
- Survey ResultsThe Future of AI in IT OperationsGet the Results
What’s holding organizations back from implementing automation and AI in their IT operations?
- Learn
- Main Menu
- Learn
Learn
Catalyze and automate essential operations throughout the organization with these insights.
- Blog
- Community
- Resources
- Events
- Podcasts
- Platform Tours
- Customer Success Stories
- Training & Certification
- Explore All Resources
- 157% Return on InvestmentForrester TEI ReportRead the Report
Forrester examined four enterprises running large, complex IT estates to see the results of an investment in ScienceLogic’s SL1 AIOps platform.
- Company
- Main Menu
- Company
Company
We’re on a mission to make your IT team’s lives easier and your customers happier.
- About Us
- Careers
- Newsroom
- Leadership
- Contact Us
- Congratulations2024 Innovators AwardsView the Winners
See how this year’s winners have demonstrated exceptional creativity and set new standards in leveraging the ScienceLogic AI Platform to solve complex IT Ops challenges.
Comprehensive Observability: Key Performance Metrics to Monitor in Cloud Environments
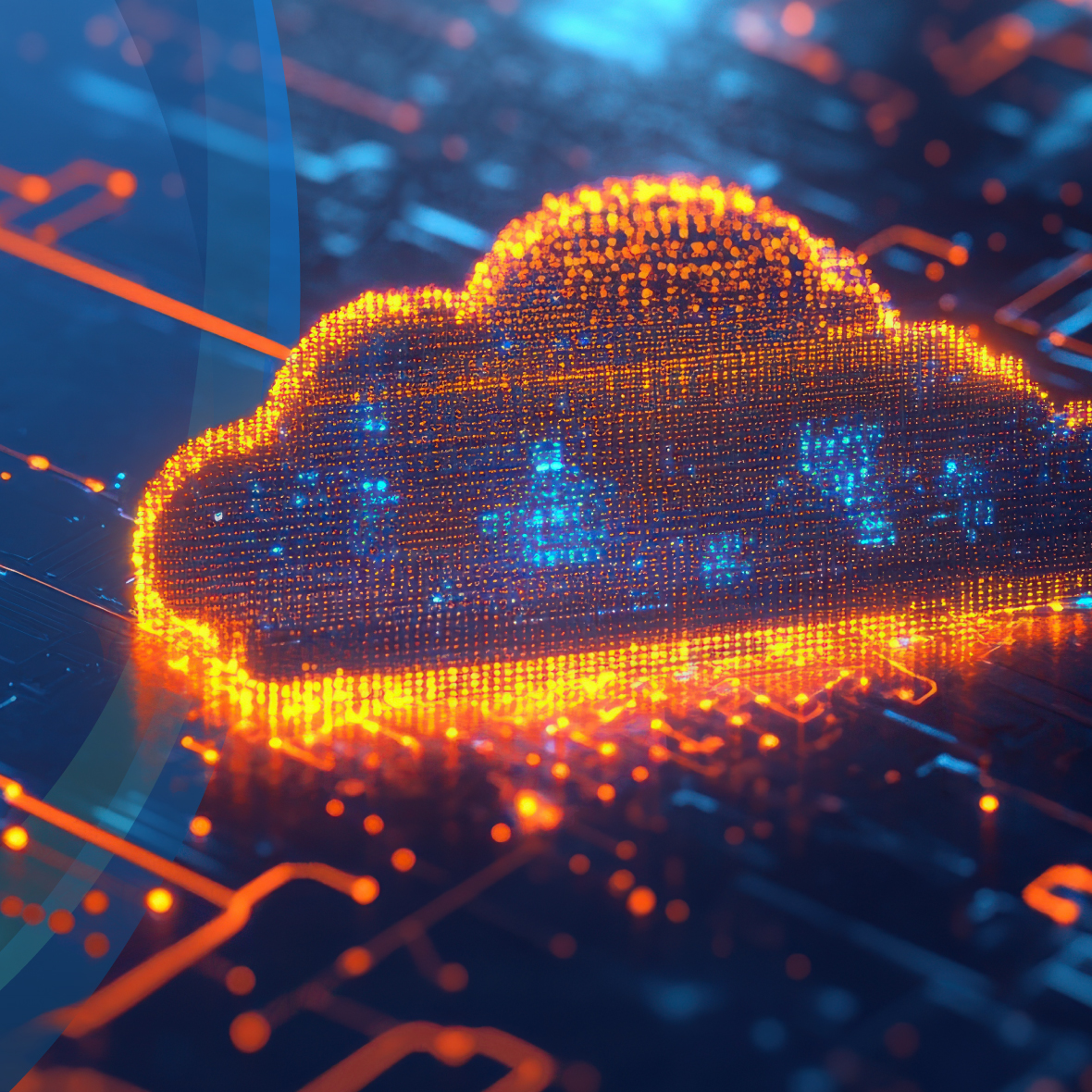
Enterprises need strong observability to ensure system reliability, proactively detect and resolve issues, optimize performance, enhance security, and maintain seamless business operations across complex distributed environments.
The observability, insights and telemetry, like those offered by the ScienceLogic AI Platform and Skylar AI suite of services, can assist businesses in identifying cost savings, informing tool consolidation, and supporting a flexible cloud infrastructure capable of supporting emerging technologies. However, it’s important to consider other factors that can impact cloud cost optimization, such as performance.
Similar to how availability and reliability metrics illustrate the status of an IT environment’s operations, performance also delivers a critical overview of operations. Monitoring performance metrics in ITOps allows teams to gain valuable insights into the health, stability, and efficiency of their IT infrastructure.
ScienceLogic monitors many key performance metrics to ensure IT optimization, some of which include:
Performance Metrics
- Latency
Latency refers to the time, measured in milliseconds, it takes for data to transfer across the network to a device. High latency or lag can slow down cloud-based application response times and hinder the ability to meet computing demands, particularly in data-intensive transactions. This can lead to poor user experience and even service failure. Many organizations mistakenly attribute latency to network issues and invest in new network circuits, which drives up costs without pinpointing the root cause.
ScienceLogic measures latency by evaluating a device’s ability to accept connections and data from the network. This enables better identification of problematic devices. The platform also generates event alerts and guides potential corrective actions.
- Throughput
Throughput measures the rate at which data is processed and transmitted through a cloud service, usually expressed as megabytes per second (Mbps). Higher throughput results in faster data processing and more responsive applications, enabling businesses to handle more transactions simultaneously. Throughput can be affected by network performance, resource allocation, data volumes, and the nature of requests.
Throughput metrics to observe include average task time taken to process data, bandwidth usage, data transfer rate, IOPS (Input/Output Operations Per Second), requests per minute, disk throughput, and more.
- Error Rates
Error rates in cloud applications and services indicate the health and reliability of cloud infrastructure. Errors can be server-side (failed requests) or client-side (how users interact with an application). A low error rate indicates the system is stable and can process requests successfully. High error rates, typified by bugs in code, misconfigurations, or resource limitations, can lead to poor user experiences.
ScienceLogic tracks error rates and other KPIs in real-time, making it easy to spot abnormal patterns and anomalies before these become problems, automatically identify root cause, and alert ITOps teams to unusual activity. The ScienceLogic AI Platform continuously monitors system performance and can recognize known and unknown errors to prevent downtime or outages or system disruptions proactively.
- CPU Usage
CPU usage measures whether a CPU is underutilized, overutilized, or optimally utilized. High CPU usage or workloads can slow system response times and impact application performance. Understanding CPU usage can help with resource allocation and cost optimization. For example, resource-intensive workloads can be dynamically scaled up or down based on demand to improve overall system performance without incurring additional costs.
ScienceLogic measures CPU utilization as a percentage per device. If a device contains multiple CPUs, the report displays the total combined CPU usage in percentage.
- Memory Utilization
High memory utilization by cloud-based systems can cause performance issues, bottlenecks, and even downtime. Techniques for monitoring available memory resources and consumption include monitoring total memory usage per device and average memory usage over time. Some trends to look for include sustained periods of high memory usage (close to 100%), which may indicate the device needs more memory resources to handle its workload efficiently. On the other hand, sudden spikes or drops can be caused by application behavior or configuration changes.
- Storage Utilization
Storage utilization refers to the amount of storage space used compared to the total available storage capacity. Monitoring this metric is crucial for ensuring that applications have enough storage resources to operate efficiently and for preventing potential issues related to storage shortages.
Metrics to track storage capacity and usage trends include total storage capacity, used storage, available storage, and utilization percentage.
Performance Metric Monitoring for Optimized Investments
These performance metrics serve only as a snapshot of the comprehensive data and context the ScienceLogic AI Platform collects and considers.
With the insights and context unlocked by monitoring performance metrics, ScienceLogic can help clients ensure their IT investments deliver maximum value. Monitoring these metrics enables data-driven decisions, preventing over- and under-investing and ensuring that every dollar spent on technology translates into measurable performance improvements, enhanced user experience, and long-term operational success.
Why Cloud Cost Optimization Has Come to the Fore
The cloud was once seen as a cost-effective way to manage IT infrastructure. However, the move to using multiple cloud providers and increasing costs of generative AI and large language models (LLMs) hosted in the cloud significantly affect OpEx budgets. Gartner forecasts that worldwide public cloud spending will surpass $675 billion this year. That’s a year-over-year growth of more than 20% spurred by Gen AI-enabled applications.
This complex ecosystem has also led to tool sprawl, where different teams use different tool sets to monitor cloud performance. This has resulted in complex and expensive integrations, as well as increased maintenance, licensing, and update costs.
And for every additional dollar spent on cloud infrastructure, less is spent on innovation and propelling the business forward.
Observability is the key to addressing these challenges and more. With cloud cost optimization an increasing priority for customers, ScienceLogic helps them achieve the necessary level of observability and context with the ScienceLogic AI Platform.
To learn more about how the ScienceLogic AI Platform and Skylar suite of advanced AI capabilities can help capture and contextualize key performance, user experience and reliability metrics for cloud cost optimization, visit: https://sciencelogic.com/platform/skylar-analytics
Transform IT Problem-Solving
Skylar Advisor harnesses the power of artificial intelligence to deliver critical insights, predictive analytics, and targeted recommendations in real-time. Its institutional knowledge empowers users of all skill levels to swiftly resolve complex IT challenges with plain-language explanations of failures and solutions.