- Why ScienceLogic
- Main Menu
- Why ScienceLogic
Why ScienceLogic
See why our AI Platform fuels innovation for top-tier organizations.
- Why ScienceLogic
- Customer Enablement
- Trust Center
- Technology Partners
- Pricing
- Contact Us
- Product ToursSee ScienceLogic in actionTake a Tour
Experience the platform and use cases first-hand.
- Platform
- Main Menu
- Platform
Platform
Simplified. Modular-based. Efficient. AI-Enabled.
- Platform Modules
- Core Technologies
- Platform Overview
- Virtual ExperienceSkylar AI RoadmapView Now
Learn about our game-changing AI innovations! Join this virtual experience with our CEO, Dave Link and our Chief Product Officer, Mike Nappi.
- Solutions
- Main Menu
- Solutions
Solutions
From automating workflows to reducing MTTR, there's a solution for your use case.
- By Industry
- By Use Case
- By Initiative
- Explore All Solutions
- Survey ResultsThe Future of AI in IT OperationsGet the Results
What’s holding organizations back from implementing automation and AI in their IT operations?
- Learn
- Main Menu
- Learn
Learn
Catalyze and automate essential operations throughout the organization with these insights.
- Blog
- Community
- Resources
- Events
- Podcasts
- Platform Tours
- Customer Success Stories
- Training & Certification
- Explore All Resources
- 157% Return on InvestmentForrester TEI ReportRead the Report
Forrester examined four enterprises running large, complex IT estates to see the results of an investment in ScienceLogic’s SL1 AIOps platform.
- Company
- Main Menu
- Company
Company
We’re on a mission to make your IT team’s lives easier and your customers happier.
- About Us
- Careers
- Newsroom
- Leadership
- Contact Us
- Congratulations2024 Innovators AwardsView the Winners
See how this year’s winners have demonstrated exceptional creativity and set new standards in leveraging the ScienceLogic AI Platform to solve complex IT Ops challenges.
Understanding the Observability Data Lifecycle: From Data Ingestion to Automated Actions
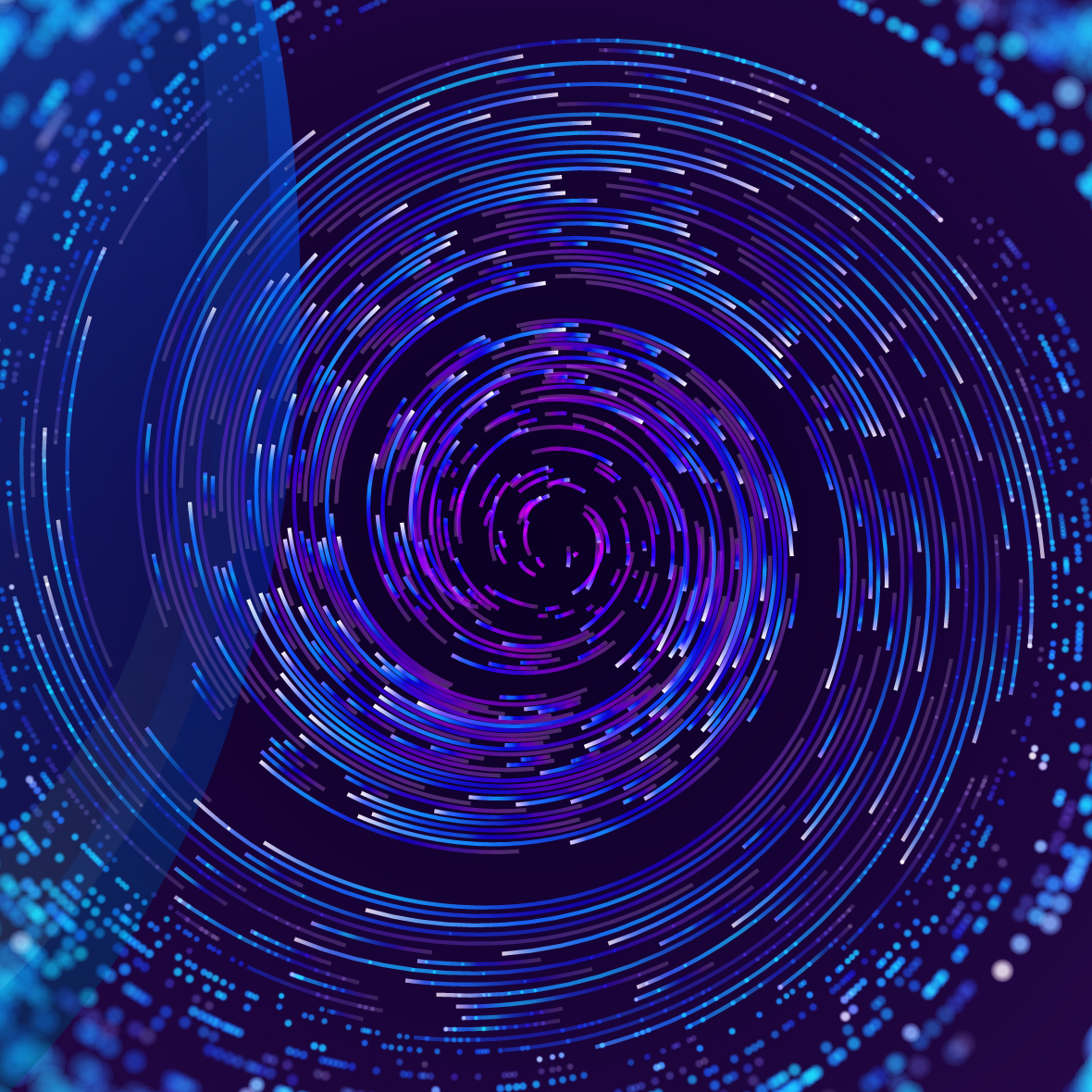
Modern IT estates are increasingly complex, generating vast amounts of data – some critical and actionable, but much of it mere noise. Extracting meaningful insights to ensure optimal system health and IT performance is beyond the scope of humans.
This is where observability, enhanced by AI and automation, becomes essential.
When combined, this modern approach to AIOps cuts through the noise, leveraging advanced analytics and automation to identify actionable insights and drive intelligent, proactive responses. By integrating these technologies, organizations can optimize performance across their hybrid environments, work at the speed today’s businesses demand, drive innovation, and improve customer experiences.
The Importance of Hybrid Observability Powered by AI in Modern IT Environments
IT management powered by AI is essential for ITOps teams to navigate today’s complex IT environments by providing improved efficiency, real-time insights, and automation.
Hybrid observability supported by AI tackles the complexities of multi-dimensional data flows and the intricate dependencies between technologies. It achieves this by building context-rich data lakes that encompass the entire application stack. This approach minimizes noise in performance management systems and provides valuable insights that support automation and enable rapid resolution times.
The main benefit of this approach is that it gives IT teams the speed and agility they need to detect incidents early, ensure the uptime of critical services, and deliver an optimal digital customer experience.
With hybrid observability and AI, ITOps professionals can:
- Assess business impact when an incident occurs and prioritize based on business relevance.
- Diagnose root cause and identify future problems.
- Automate incident resolution.
- Automate IT workflows.
However, to achieve these benefits, organizations must first understand and master the observability data lifecycle.
The observability data lifecycle encompasses the processes through which data is collected, processed, analyzed, and acted upon to gain insights into the health, performance, and reliability of systems.
The Observability Data Lifecycle: An Overview
The observability data lifecycle is complex, and mastering it is vital for organizations that aim to enhance efficiency, minimize downtime, and provide exceptional user experiences.
Let’s look at each stage of the observability data lifecycle and why each is critical to streamlining IT operations and driving efficiencies across the enterprise.
1. Data Ingestion: The Foundation
Data ingestion refers to the process of gathering data from various sources (logs, metrics, events, traces, and more) within the IT infrastructure and consolidating it into a data lake in real-time for complete visibility into the environment. This ingestion process is made possible by hybrid observability where telemetry is ingested into a platform to understand the health, performance, and behavior of applications, services, and infrastructure.
Traditional AIOps platforms take a data-agnostic approach to data ingestion. Relying on teams of data scientists to collect, clean, and organize large bodies of data before they can make sense of it. However, most organizations don’t have access to a team of data scientists or the time and resources to ingest data in this way.
The better method is a data-aware approach. For example, the ScienceLogic AI Platform – which leverages hybrid observability powered by AI – automatically converges log and metrics data from isolated systems into a contextualized data lake for more meaningful analysis, coordination, and enhanced decision-making – without requiring a team of data scientists to clean and structure the data before applying analytics.
2. Data Processing: Transforming Raw Data
By converging data from across the entire IT estate, ScienceLogic then leverages ML algorithms to filter out irrelevant information, effectively reducing noise and homing in on the most relevant signals.
The ScienceLogic AI Platform also normalizes data into a standard format and enriches raw data with contextual information such as device relationships, location, and business impacts. This approach provides a more complete picture of the IT environment. Plus, by building context, ScienceLogic aligns technical insights with business outcomes, ensuring that teams focus on the highest-priority incidents.
In addition, Skylar Analytics, a key component of the ScienceLogic suite of advanced AI capabilities, layers in advanced AI/ML analytics for greater efficiency and accuracy in deep data exploration and impactful visualizations so IT can monitor and manage system health more effectively.
3. Pattern Recognition and Anomaly Detection
Guided by engineers’ actions and historical and diagnostic data, the ScienceLogic AI Platform can recognize recurring operational patterns in metrics, logs, and events, such as periodic spikes in resource usage or frequent bottlenecks. ML models can also identify trends and baseline behaviors, assisting engineers in understanding normal operational behavior as well as anomalies and deviations – in real-time.
Patterns and anomalies are correlated across the IT ecosystem so ITOps teams can determine the significance of an issue, its business impact, and take proactive remediation action.
Common techniques used in this practice include clustering, time-series analysis, and supervised learning.
4. Root Cause Analysis: Pinpointing Issues
With a data-aware approach to AIOps, IT teams can now begin to automate root-cause analysis (RCA).
RCA plays a pivotal role in problem resolution by helping minimize downtime, improve service quality, and enhance operational efficiency.
ScienceLogic’s Skylar Automated RCA employs a multifaceted approach to RCA, using advanced methodologies such as dependency mapping, causality inference, and historical analysis to accurately identify and resolve the underlying causes of IT issues.
For example, if a database failure occurs, Skylar Automated RCA reveals which applications or services rely on the database, helping to isolate the problem quickly. Meanwhile, causality analyzes the events leading up to an incident and identifies the responsible system. Historical analysis uses past data to identify trends, patterns, or recurring issues.
The benefits of this approach include:
- Issues are diagnosed 10X faster.
- Critical unknown unknowns are identified before they cause incidents.
- Users can understand what is happening in their environment, even if they don’t speak ‘log.’
- ITOps teams receive recommendations and goal-driven actions, guided by agentic AI.
5. Predictive Analysis: Staying Ahead of Problems
Centralized and contextualized data can also be used to train ML models to predict and detect future issues. Leveraging historical data, ScienceLogic goes beyond observability to provide AI-driven actions, such as predicting outages, performance degradation, and capacity needs, so engineers can move from reactive troubleshooting to proactive management.
These findings and recommendations can be presented in human-friendly language with an agentic AI assistant tool, such as Sklyar Advisor, which is designed to enhance operations by providing intelligent, adaptive, and proactive capabilities, including:
- Context-rich insights.
- Actionable recommendations: Skylar Advisor identifies potential issues and suggests specific actions to prevent or resolve them. It also provides optimization guidance and integrates with the ScienceLogic platform to execute recommended actions seamlessly.
- Prevent issues before they occur and optimize resources.
6. Automated Actions: The End Goal
A data-aware approach to AIOps is the only way to achieve true automation, at scale.
In addition to intelligent RCA, with ScienceLogic, IT teams can craft automated workflows and analyses such as incident management, change management, configuration management, and self-healing, auto-scaling, Autonomic IT environment for the autonomous business.
Importantly, autonomy doesn’t mean giving up control. Any agentic AI solution must strike a balance between automation and human oversight. For instance, ScienceLogic enables humans to guide the AI by incorporating tools, processes, and features that allow IT teams to control and fine-tune how unsupervised AI and ML are applied in IT operations. This collaboration ensures that agentic AI delivers actionable, reliable outcomes aligned with organizational needs.
By handling the heavy lifting of IT operations, ScienceLogic empowers organizations to transform their IT teams from support centers into innovation hubs.
Getting Started with AIOps
The observability data lifecycle is a journey that requires strategic planning and a strong business case. Ready to learn how your organization can progress from coordinated data ingestion to automated actions and Autonomic IT? Contact us today to start leveraging hybrid observability powered by AI to transform your IT operations.
eBook: The Future of AI in IT Operations
Learn how organizations like yours are implementing AI and Automation into their IT operations.