- Why ScienceLogic
- Main Menu
- Why ScienceLogic
Why ScienceLogic
See why our AI Platform fuels innovation for top-tier organizations.
- Why ScienceLogic
- Customer Enablement
- Trust Center
- Technology Partners
- Pricing
- Contact Us
- Product ToursSee ScienceLogic in actionTake a Tour
Experience the platform and use cases first-hand.
- Platform
- Main Menu
- Platform
Platform
Simplified. Modular-based. Efficient. AI-Enabled.
- Platform Modules
- Core Technologies
- Platform Overview
- DemoExperience the Power of ScienceLogicRegister Now
See how today’s technology leaders are reducing costs, streamlining IT operations, and improving the customer experience.
- Solutions
- Main Menu
- Solutions
Solutions
From automating workflows to reducing MTTR, there's a solution for your use case.
- By Industry
- By Use Case
- By Initiative
- Explore All Solutions
- Survey ResultsThe Future of AI in IT OperationsGet the Results
What’s holding organizations back from implementing automation and AI in their IT operations?
- Learn
- Main Menu
- Learn
Learn
Catalyze and automate essential operations throughout the organization with these insights.
- Blog
- Community
- Resources
- Events
- Podcasts
- Platform Tours
- Customer Success Stories
- Training & Certification
- Explore All Resources
- Upcoming WebinarStack the Deck in Your Favor: Automation for MSP ExcellenceRegister Today
From predictive analytics to auto-remediation, learn how to turn a full house of IT problems into a royal flush of solutions in this live webinar.
April 30, 2025
- Company
- Main Menu
- Company
Company
We’re on a mission to make your IT team’s lives easier and your customers happier.
- About Us
- Careers
- Newsroom
- Leadership
- Contact Us
- Congratulations2024 Innovators AwardsView the Winners
See how this year’s winners have demonstrated exceptional creativity and set new standards in leveraging the ScienceLogic AI Platform to solve complex IT Ops challenges.
Why Full AI-Stack Visibility is Key to High-Performing GPUs and AI Models
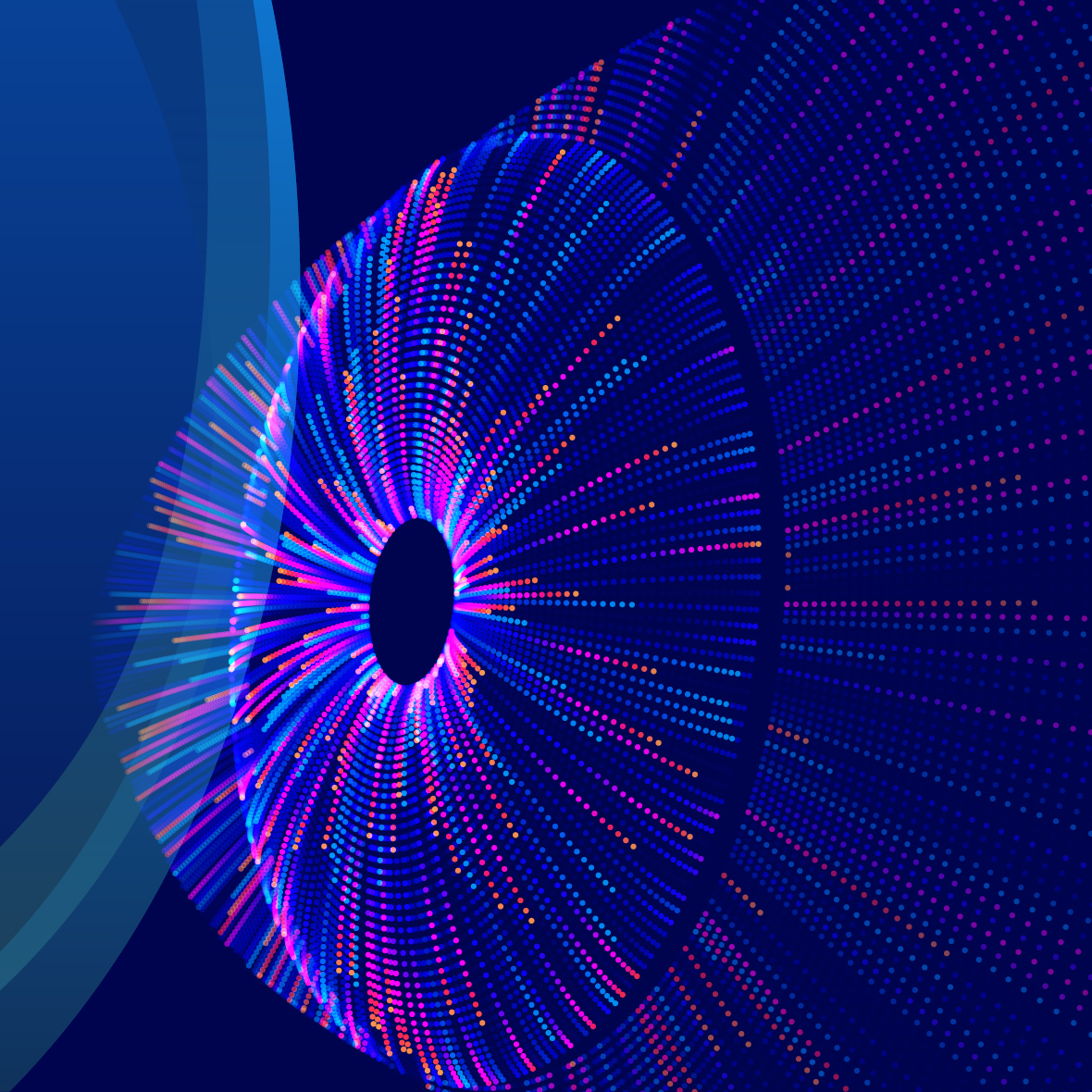
Why Full AI-Stack Visibility is Key to High-Performing GPUs and AI Models
The generative AI market is poised to explode. From AI-based co-pilots and assistants to new use cases across healthcare, marketing, sales, software development, and more, generative AI is unleashing a new wave of productivity, efficiency, and transformative employee and customer experiences.
The growing need for AI capabilities is evident with the market’s investment in AI infrastructure, which includes vital components such as servers, graphics processing units (GPUs), neural processing units (NPUs), operating systems, Kubernetes workers, containers, and more. By some predictions, AI server spending will reach $80 billion by 2027. Adjunct to this spending is the investment in the AI technology stack that includes the framework and foundational model components of the AI workload.
However, this complex, multi-layered AI architecture challenges CIOs and ITOps teams, particularly at the intersection of supporting AI infrastructure and AI model monitoring and management.
AI infrastructure vs. AI workload: The challenge of siloed tech stacks
As generative AI technologies continue gaining momentum, organizations must ensure these investments are high-performing and deliver value.
The challenge lies in how IT teams can oversee the performance of both AI infrastructure (particularly GPUs and NPUs) and AI workloads (such as LLM frameworks), which have traditionally been managed separately.
This lack of integration arises because current AI workloads are highly customized for specific use cases and organizational needs – an approach that will likely continue for at least another three to five years as AI workload technologies evolve and become more standardized.
With so many moving parts across disparate tech stacks and hosted environments – cloud and on-premises – and many tools needed to monitor and manage them, ITOps teams often struggle to achieve comprehensive service visibility and unified insights into both infrastructure components and the health of the software running on that infrastructure. This complexity makes it difficult to pinpoint issues and keep model performance in check.
The GPU/NPU monitoring dilemma
Traditional monitoring solutions fall short for another reason: the GPU/NPU monitoring dilemma.
GPUs and NPUs are essential for tasks like training data sets, making inferences, and managing graphics-heavy workloads. However, they also bring heightened complexity and risk. With the industry experiencing a high frequency of GPU-based server failure rates, there is a critical need for precise visibility and insights into system health.
This dilemma is exacerbated by the fact that organizations are increasingly using on-premises GPU infrastructure for to process their LLMs. This places a significant burden on engineers who must ensure all assets, systems, and processes across the AI architecture are aligned and work together seamlessly.
To ensure the reliability and peak performance of GPUs and NPUs, engineers urgently require comprehensive data on service health. This includes monitoring utilization levels – optimal performance typically peaks around 70% to prevent overheating – alongside metrics for query volume, power consumption, and other relevant factors.
Global, full-stack visibility and insights are key
As organizations integrate generative AI into their crucial operations, they need a comprehensive monitoring and management strategy that goes beyond isolated tools and siloed monitoring.
They require a solution that offers global visibility and full-stack monitoring, including real-time, accurate root cause analysis across both AI infrastructure and workloads. This comprehensive approach will enable ITOps teams to understand the underlying causes of issues, rather than merely addressing their symptoms. By adopting this proactive strategy, organizations can achieve a more stable, resilient, and high-performing AI architecture.
ScienceLogic is the answer to this monumental challenge.
ScienceLogic: Blending AI infrastructure and AI workload monitoring
No matter how complex the overall AI architecture is, ScienceLogic’s suite of advanced AI capabilities – Skylar AI – drastically reduces visibility gaps and provides a single source of truth in AI infrastructure and AI workload monitoring, on-premises and in the cloud.
Skylar AI ingests telemetry from across the multi-layered AI tech stack, including servers, containers, operating systems, and switches. It uses best-in-the-breed analytical and AI/ML algorithms to proactively uncover insights, curate data, and provide a holistic view of the stack, automatically guiding users to business-impacting issues before they happen.
For example, if an issue arises that causes a spike in latency or a GPU overheats due to over-utilization, ITOps teams can use Skylar Automated RCA to move beyond simple incident alerts and diagnose the root cause automatically, quickly and in real-time. It also suggests recommended actions, saving hours (sometimes days) of manual effort.
These same capabilities can also be extended to previously siloed AI workloads, enabling enterprises to gain a holistic view and control of their investments.
Advancing the AIOps conversation
By monitoring the entire AI stack, ScienceLogic is advancing the AIOps conversation. Moving beyond siloed monitoring to reduce the complexity of developing, deploying, and monitoring generative AI, accelerate AI adoption, and future-proof those investments.
Contact us to learn how ScienceLogic enables full-stack AI architecture monitoring.